Lower Columbia River Fish Population Indexing Analysis 2014
The suggested citation for this analytic report is:
Thorley, J.L. and Hogan, P.M. (2015) Lower Columbia River Fish Population Indexing Analysis 2014. A Poisson Consulting Analysis Report. URL: https://www.poissonconsulting.ca/f/1057520643.
Background
In the mid 1990s BC Hydro began operating Hugh L. Keenleyside (HLK) Dam to reduce dewatering of Mountain Whitefish and Rainbow Trout eggs.
The primary goal of the Lower Columbia River Fish Population Indexing program is to answer two key management questions:
What are the abundance, growth rate, survival rate, body condition, age distribution, and spatial distribution of subadult and adult Whitefish, Rainbow Trout, and Walleye in the Lower Columbia River?
What is the effect of inter-annual variability in the Whitefish and Rainbow Trout flow regimes on the abundance, growth rate, survival rate, body condition, and spatial distribution of subadult and adult Whitefish, Rainbow Trout, and Walleye in the Lower Columbia River?
The inter-annual variability in the Whitefish and Rainbow Trout flow regimes was quantified in terms of the percent egg dewatering as greater flow variability is associated with more egg stranding.
Methods
Data Preparation
The fish indexing data were provided by Golder Associates in the form of an Access database. The discharge and temperature data were queried from a BC Hydro database maintained by Poisson Consulting. The Rainbow Trout egg dewatering estimates were provided by Irvine et al (2015) and the Mountain Whitefish egg stranding estimates by BC Hydro.
The data were prepared for analysis using R version 3.2.1 (R Core Team 2014).
Statistical Analysis
Hierarchical Bayesian models were fitted to the fish indexing data using R version 3.2.1 (Team 2013) and JAGS 3.4.0 (Plummer 2012) which interfaced with each other via jaggernaut 2.3.1 (Thorley 2013). For additional information on hierarchical Bayesian modelling in the BUGS language, of which JAGS uses a dialect, the reader is referred to Kery and Schaub (2011, 41–44).
Unless specified, the models assumed vague (low information) prior distributions (Kery and Schaub 2011, 36). The posterior distributions were estimated from a minimum of 1,000 Markov Chain Monte Carlo (MCMC) samples thinned from the second halves of three chains of at least 1,000 iterations in length (Kery and Schaub 2011, 38–40). Model convergence was confirmed by ensuring that Rhat (Kery and Schaub 2011, 40) was less than 1.1 for each of the parameters in the model (Kery and Schaub 2011, 61). Model adequacy was confirmed by examination of residual plots.
The posterior distributions of the fixed (Kery and Schaub 2011, 75) parameters are summarised in terms of a point estimate (mean), lower and upper 95% credible limits (2.5th and 97.5th percentiles), the standard deviation (SD), percent relative error (half the 95% credible interval as a percent of the point estimate) and significance (Kery and Schaub 2011, 37, 42).
In general variable selection was achieved by dropping insignificant (Kery and Schaub 2011, 37, 42) fixed (Kery and Schaub 2011, 77–82) variables and uninformative random variables. A fixed variable was considered to be insignificant if its significance was \(\geq\) 0.05 while a random variable was considered to be uninformative if its percent relative error was \(\geq\) 80%.
The results are displayed graphically by plotting the modelled relationships between particular variables and the response with 95% credible intervals (CRIs) with the remaining variables held constant. In general, continuous and discrete fixed variables are held constant at their mean and first level values respectively while random variables are held constant at their typical values (expected values of the underlying hyperdistributions) (Kery and Schaub 2011, 77–82). Where informative the influence of particular variables is expressed in terms of the effect size (i.e., percent change in the response variable) with 95% CRIs (Bradford, Korman, and Higgins 2005).
Condition
The expected weight of fish of a given length were estimated from the data using a mass-length model (He et al. 2008). Key assumptions of the condition model include:
- The expected weight is allowed to vary with length and date.
- The expected weight is allowed to vary randomly with year.
- The relationship between weight and length is allowed to vary with date.
- The relationship between weight and length is allowed to vary randomly with year.
- The residual variation in weight is log-normally distributed.
Only previously untagged fish were included in models to avoid potential effects of tagging on body condition. Preliminary analyses indicated that the annual variation in weight was not correlated with the annual variation in the relationship between weight and length.
Growth
Annual growth of fish were estimated from the inter-annual recaptures using the Fabens method (Fabens 1965) for estimating the von Bertalanffy (VB) growth curve (von Bertalanffy 1938). The VB curves is based on the premise that:
\[ \frac{\text{d}L}{\text{d}t} = k (L_{\infty} - L)\]
where \(L\) is the length of the individual, \(k\) is the growth coefficient and \(L_{\infty}\) is the mean maximum length.
Integrating the above equation gives:
\[ L_t = L_{\infty} (1 - e^{-k(t - t_0)})\]
where \(L_t\) is the length at time \(t\) and \(t_0\) is the time at which the individual would have had no length.
The Fabens form allows
\[ L_r = L_c + (L_{\infty} - L_c) (1 - e^{-kT})\]
where \(L_r\) is the length at recapture, \(L_c\) is the length at capture and \(T\) is the time between capture and recapture.
Key assumptions of the growth model include:
- The mean maximum length \(L_{\infty}\) is constant.
- The growth coefficient \(k\) is allowed to vary randomly with year.
- The residual variation in growth is normally distributed.
Length-At-Age
The expected length-at-age of Mountain Whitefish and Rainbow Trout were estimated from annual length-frequency distributions using a finite mixture distribution model (Macdonald and Pitcher 1979). Key assumptions of the length-at-age model include:
- There are three distinguishable age-classes for each species: Age-0, Age-1 and Age-2+.
- The proportion of fish in each age-class is allowed to vary randomly with year.
- The expected growth between age-classes is allowed to vary with age-class.
- The expected growth between age-classes is allowed to vary randomly with age-class within year.
- The expected length increases with age-class.
- The expected length is allowed to vary with year within age-class.
- The expected length is allowed to vary as a second-order polynomial with date.
- The relationship between length and date is allowed to vary randomly with age-class.
- The residual variation in length is normally distributed.
- The standard deviation of this normal distribution is allowed to vary randomly with age-class.
The model was used to estimate the cut-offs between age-classes by year. For the purposes of estimating other population parameters by age-class, Age-0 individuals were classified as fry, Age-1 individuals were classified as subadult, and Age-2+ individuals were classified as adult. Walleye could not be separated by life stage due to a lack of discrete modes in the length-frequency distributions for this species. Consequently, all captured Walleye were considered to be adults.
The results include plots of the age-class density for each year by length as predicted by the length-at-age model. Density is a measure of relative frequency for continuous values.
Observer Length Correction
The bias (accuracy) and error (precision) in observer’s fish length estimates were quantified using a model with a categorical distribution which compared the proportions of fish in different length-classes for each observer to the equivalent proportions for the measured fish. Key assumptions of the observer length correction model include:
- The proportion of fish in each length-class is allowed to vary with year.
- The expected length bias is allowed to vary with observer.
- The expected length error is allowed to vary with observer.
- The expected length bias and error for a given observer does not vary by year.
- The residual variation in length is normally distributed.
The observed fish lengths were corrected for the estimated length biases before being classified as fry, subadult and adult based on the length-at-age cutoffs.
Survival
The annual survival rate was estimated by fitting a Cormack-Jolly-Seber model (Kery and Schaub 2011, 172–77) to inter-annual recaptures.
Key assumptions of the survival model include:
- Survival is constant for subadults.
- Survival varies randomly with year for adults.
- The encounter probability for adults is allowed to vary with the total bank length sampled.
Site Fidelity
The extent to which sites are closed, i.e., fish remain at the same site between sessions, was evaluated with a logistic ANCOVA (Kery 2010). The model estimates the probability that intra-annual recaptures were caught at the same site versus a different one. Key assumptions of the site fidelity model include:
- The expected site fidelity is allowed to vary with fish length.
- Observed site fidelity is Bernoulli distributed.
Length as a second-order polynomial was not found to be a significant predictor for site fidelity.
Capture Efficiency
The probability of capture was estimated using a recapture-based binomial model (Kery and Schaub 2011, 134–36, 384–88).
Key assumptions of the capture efficiency model include:
- The capture probability varies randomly by session within year.
- The probability of a marked fish remaining at a site is the estimated site fidelity.
- The number of recaptures is described by a binomial distribution.
Abundance
The abundance was estimated from the catch and bias-corrected observer count data using an overdispersed Poisson model (Kery and Schaub 2011, 55–56). The model assumed that the capture efficiency was the mean estimate from the capture efficiency model and that the number of observed fish was a multiple of the number of captured fish. The annual abundance estimates represent the total number of fish in the study area.
Key assumptions of the abundance model include:
- The capture efficiency is the mean estimate from the capture efficiency model.
- The observer efficiency varies from the capture efficiency.
- The lineal fish density varies randomly with site, year and site within year.
- The catches and counts are described by a Poisson-gamma distribution.
The annual distribution of each species was calculated using the shannon index of evenness (\(E\)) where \(S\) was the number of sites and \(p_i\) the proportion of the population belonging to the ith site.
\[ E = \frac{-\sum p_i \log(p_i)}{ln(S)}\]
Long-Term Trends
Trends common to the fish index and environmental annual time series were identified using dynamic factor analysis (DFA) (Zuur, Tuck, and Bailey 2003) – a dimension-reduction technique designed for time-series data.
The fish index time series were the condition (Con), growth (Grw) length-at-age (Len), survival (Sur) and Abundance (Abn) by species (MW = Mountain Whitefish, RB = Rainbow Trout, WP = Walleye) and life stage (Sub = Subadult, Ad = Adult) or age (Age0, Age1).
The environmental time series were the mean (DisMe) and average hourly absolute difference (DisDi) in discharge at Birchbank, the average water temperature (TemMe) at Norns Creek by quarterly period and the annual proportional egg loss through dewatering (Regime) by species (MW = Mountain Whitefish, RB = Rainbow Trout).
The average hourly absolute discharge difference was calculated by differencing the mean hourly discharge time series and taking the average of the absolute differences. Mathematically this is equivalent to: \[ \frac{\sum |x_{1}-x_{2}| + |x_{2}-x_{3}| + ... + |x_{n-1}-x_{n}|}{n-1} \] where \(x_{1}\) is the discharge at the start of the time series and \(x_{2}\) is the discharge an hour later.
The October to December times series were lead by one year to account for the fact that they occurred after sampling and are expected to only influence the fish time series in the following year. Similarly, the Mountain Whitefish egg loss time series were also lead by one year to account for the fact that they occur over winter.
All time series were standardized prior to fitting the DFA model. Key assumptions of the model include:
- The time series are described by three underlying trends.
- The random walk processes in the trends are normally distributed.
- The residual variation in the standardised variables is normally distributed.
Due to the rotation problem the underlying trends were indeterminate (Abmann, Boysen-Hogrefe, and Pape 2014). The similarities were represented visually by using non-metric multidimensional scaling (NMDS) to map the distances onto two-dimensional space. The more similar two time series are they closer they will tend to be in the resultant NMDS plot.
Short-Term Correlations
To assess the short-term congruence between the yearly fish metrics and the environmental variables, the pair-wise distances between the residuals from the DFA model were calculated as \(1 - |\rho(x, y)|\) where \(\rho\) is the Pearson correlation and \(x\) and \(y\) are the two time series being compared.
The short-term similarities were represented visually by using NMDS to map the distances onto two-dimensional space.
Model Code
The JAGS model code, which uses a series of naming conventions, is presented below.
Condition
Variable/Parameter | Description |
---|---|
bCorrelation |
Correlation coefficient between bWeightYear and bWeightLengthYear |
bWeight |
Intercept of eLogWeight |
bWeightDayte |
Linear effect of dayte on eLogWeight |
bWeightLength |
Linear effect of length on eLogWeight |
bWeightLengthDayte |
Effect of dayte on effect of length on eLogWeight |
bWeightLengthYear[i] |
Effect of i th year on effect of length on eLogWeight |
bWeightYear[i] |
Effect of i th year on eLogWeight |
Dayte[i] |
Day of year i th fish was captured |
eLogWeight[i] |
Expected log(Weight) of i th fish |
Length[i] |
log(Length) of i th fish |
sWeight |
SD of residual variation in log(Weight) |
sWeightLengthYear |
SD of effect of year on effect of length on eLogWeight |
sWeightYear |
SD of effect of year on eLogWeight |
Weight[i] |
Observed weight of i th fish |
Year[i] |
Year i th fish was captured |
Condition - Model1
model {
bWeight ~ dnorm(5, 5^-2)
bWeightLength ~ dnorm(3, 2^-2)
bWeightDayte ~ dnorm(0, 2^-2)
bWeightLengthDayte ~ dnorm(0, 2^-2)
sWeightYear ~ dunif(0, 1)
sWeightLengthYear ~ dunif(0, 1)
for (i in 1:nYear) {
bWeightYear[i] ~ dnorm(0, sWeightYear^-2)
bWeightLengthYear[i] ~ dnorm(0, sWeightLengthYear^-2)
}
sWeight ~ dunif(0, 1)
for(i in 1:length(Length)) {
eLogWeight[i] <- bWeight
+ bWeightDayte * Dayte[i]
+ bWeightYear[Year[i]]
+ ( bWeightLength
+ bWeightLengthDayte * Dayte[i]
+ bWeightLengthYear[Year[i]]
) * Length[i]
Weight[i] ~ dlnorm(eLogWeight[i], sWeight^-2)
}
}
Growth
Variable/Parameter | Description |
---|---|
bK |
Intercept of log(eK) |
bKYear[i] |
Random effect of i th year on log(eK) |
bLinf |
Mean maximum length |
eGrowth[i] |
Expected growth between release and recapture of i th recapture |
eK[i] |
Expected von Bertalanffy growth coefficient in i th year |
Growth[i] |
Observed growth between release and recapture of i th recapture |
LengthAtRelease[i] |
Length at previous release of i th recapture |
sGrowth |
SD of residual variation in Growth |
sKYear |
SD of effect of year on log(eK) |
Year[i] |
Release year of i th recapture |
Years[i] |
Years between release and recapture of i th recapture |
Growth - Model1
model {
bK ~ dnorm (0, 5^-2)
sKYear ~ dunif (0, 5)
for (i in 1:nYear) {
bKYear[i] ~ dnorm(0, sKYear^-2)
log(eK[i]) <- bK + bKYear[i]
}
bLinf ~ dunif(100, 1000)
sGrowth ~ dunif(0, 100)
for (i in 1:length(Year)) {
eGrowth[i] <- (bLinf - LengthAtRelease[i]) * (1 - exp(-sum(eK[Year[i]:(Year[i] + Years[i] - 1)])))
Growth[i] ~ dnorm(eGrowth[i], sGrowth^-2)
}
}
Length-At-Age
Variable/Parameter | Description |
---|---|
Age[ii] |
Observed age-class of ii th fish |
bAge[ii] |
Effect of ii th age-class on logit(pAgeYear) |
bAgeYear[ii, jj] |
Effect of ii th age-class within jj th year on logit(pAgeYear) |
bDayte[ii] |
Effect of ii th age-class on linear effect of dayte on eLength |
bDayte2[ii] |
Effect of ii th age-class on quadratic effect of dayte on eLength |
bGrowthAge[ii] |
Growth of fish to ii th age-class |
bGrowthAgeYear[ii, jj] |
Growth of fish to ii th age-class within jj th year |
bLengthAgeYear[ii, jj] |
Effect of ii th age-class within jj th year on eLength |
eGrowthAgeYear[ii, jj] |
Total growth of fish to ii th age-class in jj th year |
eLength[ii] |
Expected length of ii th fish |
Length[ii] |
Observed length of ii th fish |
pAgeYear[ii, jj] |
Proportion of fish in ii th age-class within jj th year |
sAgeYear |
SD of effect of age-class within year on bAgeYear |
sGrowthAgeYear[ii] |
SD of effect of age-class within year on fish growth |
sLengthAge[ii] |
SD of residual variation in eLength of fish in ii th age-class |
Year[ii] |
Year in which ii th fish was caught |
Length-At-Age - Model1
model{
for(ii in 1:nAge){
bGrowthAge[ii] ~ dunif(10, 100)
sGrowthAgeYear[ii] ~ dunif(0, 25)
for(jj in 1:nYear) {
bGrowthAgeYear[ii, jj] ~ dnorm(0, sGrowthAgeYear[ii]^-2)
eGrowthAgeYear[ii, jj] <- bGrowthAge[ii] + bGrowthAgeYear[ii, jj]
}
}
bLengthAgeYear[1, 1] <- eGrowthAgeYear[1, 1]
for(ii in 2:nAge){
bLengthAgeYear[ii, 1] <- bLengthAgeYear[ii-1, 1] - bGrowthAgeYear[ii-1, 1] + eGrowthAgeYear[ii, 1]
}
for(jj in 2:nYear){
bLengthAgeYear[1, jj] <- eGrowthAgeYear[1, jj]
for(ii in 2:nAge){
bLengthAgeYear[ii, jj] <- bLengthAgeYear[ii-1, jj-1] + eGrowthAgeYear[ii, jj]
}
}
for(ii in 1:nAge) {
bDayte[ii] ~ dnorm(0, 10)
bDayte2[ii] ~ dnorm(0, 10)
}
sAgeYear ~ dunif(0, 5)
for(ii in 1:(nAge - 1)){
bAge[ii] ~ dnorm(0, 2^-2)
for(jj in 1:nYear){
bAgeYear[ii, jj] ~ dnorm(0, sAgeYear^-2)
}
}
for(jj in 1:nYear){
logit(pAgeYear[1, jj]) <- bAge[1] + bAgeYear[1, jj]
for(ii in 2:(nAge - 1)){
pAgeYear[ii, jj] <- (1 - sum(pAgeYear[1:ii-1, jj])) * ilogit(bAge[ii] + bAgeYear[ii, jj])
}
pAgeYear[nAge, jj] <- (1 - sum(pAgeYear[1:nAge - 1, jj]))
}
for(ii in 1:nAge){
sLengthAge[ii] ~ dunif(0, 50)
}
for(ii in 1:length(Length)){
Age[ii] ~ dcat(pAgeYear[1:nAge, Year[ii]])
eLength[ii] <- bLengthAgeYear[Age[ii], Year[ii]]
+ bDayte[Age[ii]] * Dayte[ii]
+ bDayte2[Age[ii]] * Dayte[ii]^2
Length[ii] ~ dnorm(eLength[ii], sLengthAge[Age[ii]]^-2)
}
}
Observer Length Correction
Variable/Parameter | Description |
---|---|
bLength[i] |
Relative inaccuracy of i th observer |
ClassLength[i] |
Mean length of fish belonging to i th class |
dClass[i] |
Prior value for the proportion of fish in the i th class |
eClass[i] |
Expected class of i th fish |
eLength[i] |
Expected length of i th fish |
eSLength[i] |
Expected SD of residual variation in length of i th fish |
Length[i] |
Observed fork length of i th fish |
Observer[i] |
Observer of i th fish where the first observer used a length board |
pClass[i] |
Proportion of fish in the i th class |
sLength[i] |
Relative imprecision of i th observer |
Year[i] |
Year i th fish was observed |
Observer Length Correction - Model1
model{
for(j in 1:nYear){
for(i in 1:nClass) {
dClass[i, j] <- 1
}
pClass[1:nClass, j] ~ ddirch(dClass[, j])
}
bLength[1] <- 1
sLength[1] <- 1
for(i in 2:nObserver) {
bLength[i] ~ dunif(0.5, 2)
sLength[i] ~ dunif(2, 10)
}
for(i in 1:length(Length)){
eClass[i] ~ dcat(pClass[, Year[i]])
eLength[i] <- bLength[Observer[i]] * ClassLength[eClass[i]]
eSLength[i] <- sLength[Observer[i]] * ClassSD
Length[i] ~ dnorm(eLength[i], eSLength[i]^-2)
}
}
Survival
Variable/Parameter | Description |
---|---|
bEfficiency |
Intercept for logit(eEfficiency) |
bSurvivalInterceptStage |
Intercept for logit(eSurvival) by Stage |
bSurvivalStageYear |
Effect of Year on logit(eSurvival) by Stage |
eAlive[i, j] |
Expected state (alive or dead) of ith fish in jth year |
eEfficiency[i, j] |
Expected recapture probability of ith fish in jth year |
eSurvival[i, j] |
Expected survival probability of ith fish from j-1th to jth year |
FirstYear[i] |
First year ith fish was observed |
FishYear[i, j] |
Whether ith fish was observed in jth year |
sSurvivalStageYear |
SD of effect of Year on logit(eSurvival) by Stage |
StageFishYear[i, j] |
Stage of ith fish in jth year |
Survival - Model1
model {
bEfficiency ~ dnorm(0, 5^-2)
bEfficiencySampledLength ~ dnorm(0, 5^-2)
for(i in 1:nStage) {
bSurvivalInterceptStage[i] ~ dnorm(0, 5^-2)
}
sSurvivalStageYear[1] <- 0
for(j in 1:nYear) {
bSurvivalStageYear[1,j] <- 0
}
for(i in 2:nStage) {
sSurvivalStageYear[i] ~ dunif(0, 5)
for(j in 1:nYear) {
bSurvivalStageYear[i,j] ~ dnorm (0, sSurvivalStageYear[i]^-2)
}
}
for(i in 1:nFish) {
eAlive[i, FirstYear[i]] <- 1
for(j in (FirstYear[i]+1):nYear) {
logit(eEfficiency[i,j]) <- bEfficiency + bEfficiencySampledLength * SampledLength[j]
logit(eSurvival[i,j]) <- bSurvivalInterceptStage[StageFishYear[i,j-1]] + bSurvivalStageYear[StageFishYear[i,j-1],j]
eAlive[i,j] ~ dbern(eAlive[i,j-1] * eSurvival[i,j])
FishYear[i,j] ~ dbern(eAlive[i,j] * eEfficiency[i,j])
}
}
}
Site Fidelity
Variable/Parameter | Description |
---|---|
bFidelity |
Intercept of logit(eFidelity) |
bLength |
Effect of length on logit(eFidelity) |
eFidelity[i] |
Expected site fidelity of i th recapture |
Fidelity[i] |
Whether the i th recapture was encountered at the same site as the previous encounter |
Length[i] |
Length at previous encounter of i th recapture |
Site Fidelity - Model1
model {
bFidelity ~ dnorm(0, 2^-2)
bLength ~ dnorm(0, 2^-2)
for (i in 1:length(Fidelity)) {
logit(eFidelity[i]) <- bFidelity + bLength * Length[i]
Fidelity[i] ~ dbern(eFidelity[i])
}
}
Capture Efficiency
Variable/Parameter | Description |
---|---|
bEfficiency |
Intercept for logit(eEfficiency) |
bEfficiencySessionYear |
Effect of Session within Year on logit(eEfficiency) |
eEfficiency[i] |
Expected efficiency on i th visit |
eFidelity[i] |
Expected site fidelity on i th visit |
Fidelity[i] |
Mean site fidelity on i th visit |
FidelitySD[i] |
SD of site fidelity on i th visit |
Recaptures[i] |
Number of marked fish recaught during i th visit |
sEfficiencySessionYear |
SD of effect of Session within Year on logit(eEfficiency) |
Session[i] |
Session of i th visit |
Tagged[i] |
Number of marked fish tagged prior to i th visit |
Year[i] |
Year of i th visit |
Capture Efficiency - Model1
model {
bEfficiency ~ dnorm(0, 5^-2)
sEfficiencySessionYear ~ dunif(0, 2)
for (i in 1:nSession) {
for (j in 1:nYear) {
bEfficiencySessionYear[i, j] ~ dnorm(0, sEfficiencySessionYear^-2)
}
}
for (i in 1:length(Recaptures)) {
logit(eEfficiency[i]) <- bEfficiency + bEfficiencySessionYear[Session[i], Year[i]]
eFidelity[i] ~ dnorm(Fidelity[i], FidelitySD[i]^-2) T(0, 1)
Recaptures[i] ~ dbin(eEfficiency[i] * eFidelity[i], Tagged[i])
}
}
Abundance
Variable/Parameter | Description |
---|---|
bDensity |
Intercept for log(eDensity) |
bDensitySite |
Effect of Site on log(eDensity) |
bDensitySiteYear |
Effect of Site within Year on log(eDensity) |
bDensityYear |
Effect of Year on log(eDensity) |
bVisitType |
Effect of VisitType on Efficiency |
eDensity[i] |
Expected density during ith visit |
eDispersion |
Overdispersion of Fish |
Efficiency[i] |
Survey efficiency during ith visit |
Fish[i] |
Observed count during ith visit |
ProportionSampled[i] |
Proportion of site surveyed during ith visit |
sDensitySite |
SD of effect of Site on log(eDensity) |
sDensitySiteYear |
SD of effect of Site within Year on log(eDensity) |
sDensityYear |
SD of effect of Year on log(eDensity) |
sDispersion |
SD of overdispersion term |
Site[i] |
Site of ith visit |
SiteLength[i] |
Length of site during ith visit |
VisitType[i] |
Survey type (catch versus count) during ith visit |
Year[i] |
Year of ith visit |
Abundance - Model1
model {
bDensity ~ dnorm(5, 5^-2)
bVisitType[1] <- 1
for (i in 2:nVisitType) {
bVisitType[i] ~ dunif(0, 10)
}
sDensityYear ~ dunif(0, 2)
for (i in 1:nYear) {
bDensityYear[i] ~ dnorm(0, sDensityYear^-2)
}
sDensitySite ~ dunif(0, 2)
sDensitySiteYear ~ dunif(0, 2)
for (i in 1:nSite) {
bDensitySite[i] ~ dnorm(0, sDensitySite^-2)
for (j in 1:nYear) {
bDensitySiteYear[i, j] ~ dnorm(0, sDensitySiteYear^-2)
}
}
sDispersion ~ dunif(0, 5)
for (i in 1:length(Fish)) {
log(eDensity[i]) <- bDensity + bDensitySite[Site[i]] + bDensityYear[Year[i]] + bDensitySiteYear[Site[i],Year[i]]
eDispersion[i] ~ dgamma(1 / sDispersion^2, 1 / sDispersion^2)
Fish[i] ~ dpois(eDensity[i] * SiteLength[i] * ProportionSampled[i] * Efficiency[i] * bVisitType[VisitType[i]] * eDispersion[i])
}
}
Long-Term Trends
Variable/Parameter | Description |
---|---|
bDistance[i,j] |
Euclidean distance between i th and j th Variable |
bTrendYear[t,y] |
Expected value for t th trend in y th Year |
eValue[v,y,t] |
Expected standardised value for v th Variable in y th Year considering t th trends |
sTrend |
SD in trend random walks |
sValue |
SD for residual variation in Value |
Value[i] |
Standardised value for i th data point |
Variable[i] |
Variable for i th data point |
Year[i] |
Year of i th data point |
Z[v,y] |
Expected weighting for v th Variable in y th Year |
Long-Term Trends - Model1
model{
sTrend ~ dunif(0, 1)
for (t in 1:nTrend) {
bTrendYear[t,1] ~ dunif(-1,1)
for(y in 2:nYear){
bTrendYear[t,y] ~ dnorm(bTrendYear[t,y-1], sTrend^-2)
}
}
for(v in 1:nVariable){
for(t in 1:nTrend) {
Z[v,t] ~ dunif(-1,1)
}
for(y in 1:nYear){
eValue[v,y,1] <- Z[v,1] * bTrendYear[1,y]
for(t in 2:nTrend) {
eValue[v,y,t] <- eValue[v,y,t-1] + Z[v,t] * bTrendYear[t,y]
}
}
}
sValue ~ dunif(0, 1)
for(i in 1:length(Value)) {
Value[i] ~ dnorm(eValue[Variable[i], Year[i], nTrend], sValue^-2)
}
for(i in 1:nVariable) {
for(j in 1:nVariable) {
bDistance[i,j] <- sqrt(sum((Z[i,]-Z[j,])^2))
}
}
}
Results
Model Parameters
The posterior distributions for the fixed (Kery and Schaub 2011 p. 75) parameters in each model are summarised below.
Condition - Mountain Whitefish
Parameter | Estimate | Lower | Upper | SD | Error | Significance |
---|---|---|---|---|---|---|
bWeight | 5.43202 | 5.40935 | 5.45443 | 0.01145 | 0 | 0.0010 |
bWeightDayte | -0.01408 | -0.01863 | -0.00991 | 0.00217 | 31 | 0.0010 |
bWeightLength | 3.15480 | 3.09620 | 3.20430 | 0.02700 | 2 | 0.0010 |
bWeightLengthDayte | -0.00383 | -0.01466 | 0.00810 | 0.00581 | 300 | 0.4771 |
sWeight | 0.15564 | 0.15365 | 0.15777 | 0.00103 | 1 | 0.0010 |
sWeightLengthYear | 0.11343 | 0.07683 | 0.16857 | 0.02452 | 40 | 0.0010 |
sWeightYear | 0.04737 | 0.03280 | 0.06837 | 0.00939 | 38 | 0.0010 |
Convergence | Iterations |
---|---|
1.02 | 10000 |
Condition - Rainbow Trout
Parameter | Estimate | Lower | Upper | SD | Error | Significance |
---|---|---|---|---|---|---|
bWeight | 5.93291 | 5.92213 | 5.94329 | 0.00569 | 0 | 0.001 |
bWeightDayte | -0.00396 | -0.00682 | -0.00100 | 0.00148 | 73 | 0.008 |
bWeightLength | 2.92900 | 2.89692 | 2.95973 | 0.01530 | 1 | 0.001 |
bWeightLengthDayte | 0.04578 | 0.03627 | 0.05452 | 0.00469 | 20 | 0.001 |
sWeight | 0.11152 | 0.11005 | 0.11302 | 0.00078 | 1 | 0.001 |
sWeightLengthYear | 0.05738 | 0.03662 | 0.08999 | 0.01427 | 47 | 0.001 |
sWeightYear | 0.02380 | 0.01637 | 0.03528 | 0.00488 | 40 | 0.001 |
Convergence | Iterations |
---|---|
1.02 | 10000 |
Condition - Walleye
Parameter | Estimate | Lower | Upper | SD | Error | Significance |
---|---|---|---|---|---|---|
bWeight | 6.28395 | 6.26506 | 6.30392 | 0.00997 | 0 | 0.0010 |
bWeightDayte | 0.01788 | 0.01494 | 0.02092 | 0.00153 | 17 | 0.0010 |
bWeightLength | 3.22000 | 3.16780 | 3.27360 | 0.02610 | 2 | 0.0010 |
bWeightLengthDayte | -0.01627 | -0.03562 | 0.00289 | 0.01005 | 120 | 0.0999 |
sWeight | 0.09913 | 0.09766 | 0.10066 | 0.00079 | 2 | 0.0010 |
sWeightLengthYear | 0.10466 | 0.06692 | 0.16206 | 0.02379 | 45 | 0.0010 |
sWeightYear | 0.04148 | 0.02907 | 0.06152 | 0.00844 | 39 | 0.0010 |
Convergence | Iterations |
---|---|
1.02 | 10000 |
Growth - Mountain Whitefish
Parameter | Estimate | Lower | Upper | SD | Error | Significance |
---|---|---|---|---|---|---|
bK | -1.2048 | -1.5017 | -0.9398 | 0.1467 | 23 | 7e-04 |
bLinf | 407.6700 | 401.6500 | 414.4300 | 3.2300 | 2 | 7e-04 |
sGrowth | 12.9270 | 11.6910 | 14.3740 | 0.6840 | 10 | 7e-04 |
sKYear | 0.4461 | 0.2302 | 0.8066 | 0.1451 | 65 | 7e-04 |
Convergence | Iterations |
---|---|
1.04 | 4000 |
Growth - Rainbow Trout
Parameter | Estimate | Lower | Upper | SD | Error | Significance |
---|---|---|---|---|---|---|
bK | -0.1902 | -0.2932 | -0.0613 | 0.0613 | 61 | 0.0014 |
bLinf | 500.8000 | 495.4300 | 506.3200 | 2.8000 | 1 | 0.0007 |
sGrowth | 29.7730 | 28.4550 | 31.2920 | 0.7280 | 5 | 0.0007 |
sKYear | 0.2392 | 0.1534 | 0.3665 | 0.0597 | 45 | 0.0007 |
Convergence | Iterations |
---|---|
1.04 | 1000 |
Growth - Walleye
Parameter | Estimate | Lower | Upper | SD | Error | Significance |
---|---|---|---|---|---|---|
bK | -2.7953 | -3.1163 | -2.4123 | 0.2005 | 13 | 7e-04 |
bLinf | 855.1000 | 732.9000 | 987.0000 | 72.1000 | 15 | 7e-04 |
sGrowth | 19.0060 | 17.4680 | 20.7440 | 0.8240 | 9 | 7e-04 |
sKYear | 0.3341 | 0.1829 | 0.5509 | 0.0955 | 55 | 7e-04 |
Convergence | Iterations |
---|---|
1.03 | 2000 |
Length-At-Age - Mountain Whitefish
Parameter | Estimate | Lower | Upper | SD | Error | Significance |
---|---|---|---|---|---|---|
bAge[1] | -2.0171 | -2.4440 | -1.5490 | 0.2250 | 22 | 0.0010 |
bAge[2] | -0.7937 | -1.2146 | -0.2639 | 0.2351 | 60 | 0.0078 |
bDayte[1] | 3.1560 | 2.6400 | 3.6770 | 0.2630 | 16 | 0.0010 |
bDayte[2] | 2.3960 | 1.8470 | 2.9560 | 0.2850 | 23 | 0.0010 |
bDayte[3] | 1.3680 | 0.8720 | 1.9050 | 0.2710 | 38 | 0.0010 |
bDayte2[1] | -0.7014 | -1.1140 | -0.3124 | 0.2072 | 57 | 0.0010 |
bDayte2[2] | -0.1293 | -0.5348 | 0.2649 | 0.1996 | 310 | 0.5083 |
bDayte2[3] | 1.1590 | 0.6220 | 1.6710 | 0.2710 | 45 | 0.0010 |
bGrowthAge[1] | 98.8160 | 94.9540 | 99.9690 | 1.2480 | 3 | 0.0010 |
bGrowthAge[2] | 96.4960 | 92.1720 | 99.7600 | 2.0580 | 4 | 0.0010 |
bGrowthAge[3] | 98.9290 | 95.9890 | 99.9720 | 1.0650 | 2 | 0.0010 |
sAgeYear | 0.8934 | 0.6917 | 1.1979 | 0.1277 | 28 | 0.0010 |
sGrowthAgeYear[1] | 22.2460 | 17.8750 | 24.8640 | 1.9500 | 16 | 0.0010 |
sGrowthAgeYear[2] | 9.8520 | 6.6160 | 14.8060 | 2.0880 | 42 | 0.0010 |
sGrowthAgeYear[3] | 23.9120 | 21.5990 | 24.9690 | 0.9460 | 7 | 0.0010 |
sLengthAge[1] | 14.1186 | 13.7330 | 14.5212 | 0.1964 | 3 | 0.0010 |
sLengthAge[2] | 21.1020 | 20.3580 | 21.9210 | 0.3970 | 4 | 0.0010 |
sLengthAge[3] | 45.5000 | 44.8110 | 46.2680 | 0.3800 | 2 | 0.0010 |
Convergence | Iterations |
---|---|
1.08 | 20000 |
Length-At-Age - Rainbow Trout
Parameter | Estimate | Lower | Upper | SD | Error | Significance |
---|---|---|---|---|---|---|
bAge[1] | -3.0744 | -3.3730 | -2.7692 | 0.1498 | 10 | 0.0010 |
bAge[2] | 0.4898 | 0.1597 | 0.8234 | 0.1659 | 68 | 0.0010 |
bDayte[1] | 0.8530 | 0.2570 | 1.4410 | 0.3010 | 69 | 0.0020 |
bDayte[2] | 2.9410 | 2.3720 | 3.4660 | 0.2720 | 19 | 0.0010 |
bDayte[3] | 0.2110 | -0.3830 | 0.7800 | 0.3020 | 280 | 0.4947 |
bDayte2[1] | 0.0940 | -0.4290 | 0.6170 | 0.2830 | 550 | 0.7382 |
bDayte2[2] | 0.7923 | 0.3325 | 1.2812 | 0.2461 | 60 | 0.0020 |
bDayte2[3] | 0.2540 | -0.2900 | 0.7910 | 0.2770 | 210 | 0.3421 |
bGrowthAge[1] | 99.0670 | 96.2510 | 99.9770 | 0.9690 | 2 | 0.0010 |
bGrowthAge[2] | 99.4290 | 97.8780 | 99.9810 | 0.5530 | 1 | 0.0010 |
bGrowthAge[3] | 99.5010 | 98.1120 | 99.9920 | 0.5440 | 1 | 0.0010 |
sAgeYear | 0.5897 | 0.4443 | 0.7867 | 0.0898 | 29 | 0.0010 |
sGrowthAgeYear[1] | 16.9000 | 11.6300 | 23.4300 | 3.0900 | 35 | 0.0010 |
sGrowthAgeYear[2] | 24.6750 | 23.8490 | 24.9910 | 0.3140 | 2 | 0.0010 |
sGrowthAgeYear[3] | 24.8226 | 24.3900 | 24.9951 | 0.1700 | 1 | 0.0010 |
sLengthAge[1] | 18.0360 | 17.1460 | 18.9980 | 0.4760 | 5 | 0.0010 |
sLengthAge[2] | 38.2450 | 37.5830 | 38.9190 | 0.3410 | 2 | 0.0010 |
sLengthAge[3] | 49.9233 | 49.7243 | 49.9975 | 0.0749 | 0 | 0.0010 |
Convergence | Iterations |
---|---|
1.1 | 20000 |
Observer Length Correction - Mountain Whitefish
Parameter | Estimate | Lower | Upper | SD | Error | Significance |
---|---|---|---|---|---|---|
bLength[1] | 1.00000 | 1.00000 | 1.00000 | 0.00000 | 0 | 0.001 |
bLength[2] | 0.84855 | 0.83481 | 0.86114 | 0.00681 | 2 | 0.001 |
bLength[3] | 0.79893 | 0.78635 | 0.81142 | 0.00636 | 2 | 0.001 |
bLength[4] | 0.81988 | 0.81098 | 0.84049 | 0.00680 | 2 | 0.001 |
sLength[1] | 1.00000 | 1.00000 | 1.00000 | 0.00000 | 0 | 0.001 |
sLength[2] | 3.59370 | 3.22800 | 4.01110 | 0.19890 | 11 | 0.001 |
sLength[3] | 2.02309 | 2.00081 | 2.08460 | 0.02326 | 2 | 0.001 |
sLength[4] | 2.01283 | 2.00039 | 2.04996 | 0.01354 | 1 | 0.001 |
Convergence | Iterations |
---|---|
1.01 | 40000 |
Observer Length Correction - Rainbow Trout
Parameter | Estimate | Lower | Upper | SD | Error | Significance |
---|---|---|---|---|---|---|
bLength[1] | 1.00000 | 1.00000 | 1.00000 | 0.00000 | 0 | 0.001 |
bLength[2] | 0.87327 | 0.86285 | 0.88502 | 0.00560 | 1 | 0.001 |
bLength[3] | 0.76966 | 0.74565 | 0.79334 | 0.01175 | 3 | 0.001 |
bLength[4] | 0.87359 | 0.86434 | 0.88246 | 0.00460 | 1 | 0.001 |
sLength[1] | 1.00000 | 1.00000 | 1.00000 | 0.00000 | 0 | 0.001 |
sLength[2] | 2.80700 | 2.15500 | 3.45800 | 0.33500 | 23 | 0.001 |
sLength[3] | 4.36100 | 3.17400 | 5.53300 | 0.59600 | 27 | 0.001 |
sLength[4] | 2.04270 | 2.00110 | 2.16750 | 0.04560 | 4 | 0.001 |
Convergence | Iterations |
---|---|
1.03 | 10000 |
Observer Length Correction - Walleye
Parameter | Estimate | Lower | Upper | SD | Error | Significance |
---|---|---|---|---|---|---|
bLength[1] | 1.00000 | 1.00000 | 1.00000 | 0.00000 | 0 | 0.001 |
bLength[2] | 0.91751 | 0.90139 | 0.93430 | 0.00867 | 2 | 0.001 |
bLength[3] | 0.85530 | 0.82577 | 0.89388 | 0.01776 | 4 | 0.001 |
bLength[4] | 0.92086 | 0.89945 | 0.93999 | 0.01036 | 2 | 0.001 |
sLength[1] | 1.00000 | 1.00000 | 1.00000 | 0.00000 | 0 | 0.001 |
sLength[2] | 2.70700 | 2.02200 | 4.41300 | 0.69100 | 44 | 0.001 |
sLength[3] | 2.81400 | 2.02100 | 5.06600 | 0.81400 | 54 | 0.001 |
sLength[4] | 3.18300 | 2.04500 | 4.69000 | 0.73400 | 42 | 0.001 |
Convergence | Iterations |
---|---|
1.02 | 10000 |
Survival - Mountain Whitefish
Parameter | Estimate | Lower | Upper | SD | Error | Significance |
---|---|---|---|---|---|---|
bEfficiency | -4.73400 | -6.36900 | -3.07600 | 0.88100 | 35 | 0.0010 |
bEfficiencySampledLength | 0.00772 | -0.00787 | 0.02259 | 0.00807 | 200 | 0.3390 |
bSurvivalInterceptStage[1] | -1.11500 | -1.63300 | -0.57700 | 0.26700 | 47 | 0.0010 |
bSurvivalInterceptStage[2] | 0.20800 | -0.45000 | 0.89300 | 0.33400 | 320 | 0.5292 |
sSurvivalStageYear[2] | 1.10200 | 0.51200 | 2.04700 | 0.39200 | 70 | 0.0010 |
Convergence | Iterations |
---|---|
1.06 | 80000 |
Survival - Rainbow Trout
Parameter | Estimate | Lower | Upper | SD | Error | Significance |
---|---|---|---|---|---|---|
bEfficiency | -3.12700 | -3.74300 | -2.52500 | 0.32600 | 19 | 0.0010 |
bEfficiencySampledLength | 0.00559 | 0.00009 | 0.01096 | 0.00289 | 97 | 0.0456 |
bSurvivalInterceptStage[1] | 0.08860 | -0.19640 | 0.43690 | 0.16190 | 360 | 0.6230 |
bSurvivalInterceptStage[2] | -0.47220 | -0.78580 | -0.14930 | 0.16470 | 67 | 0.0095 |
sSurvivalStageYear[2] | 0.48970 | 0.26510 | 0.84170 | 0.15310 | 59 | 0.0010 |
Convergence | Iterations |
---|---|
1.02 | 40000 |
Survival - Walleye
Parameter | Estimate | Lower | Upper | SD | Error | Significance |
---|---|---|---|---|---|---|
bEfficiency | -3.85700 | -5.53000 | -2.16200 | 0.86600 | 44 | 0.0010 |
bEfficiencySampledLength | 0.00438 | -0.00978 | 0.01852 | 0.00726 | 320 | 0.5199 |
bSurvivalInterceptStage[1] | 0.37000 | -9.13000 | 10.57000 | 4.97000 | 2700 | 0.9585 |
bSurvivalInterceptStage[2] | 0.10700 | -0.45500 | 1.02000 | 0.33100 | 690 | 0.7827 |
sSurvivalStageYear[2] | 0.65900 | 0.26500 | 1.45200 | 0.32600 | 90 | 0.0010 |
Convergence | Iterations |
---|---|
1.1 | 20000 |
Site Fidelity - Mountain Whitefish
Parameter | Estimate | Lower | Upper | SD | Error | Significance |
---|---|---|---|---|---|---|
bFidelity | -0.0300 | -0.4017 | 0.3643 | 0.1951 | 1300 | 0.8667 |
bLength | -0.0752 | -0.4663 | 0.3196 | 0.1990 | 520 | 0.6960 |
Convergence | Iterations |
---|---|
1 | 1000 |
Site Fidelity - Rainbow Trout
Parameter | Estimate | Lower | Upper | SD | Error | Significance |
---|---|---|---|---|---|---|
bFidelity | 0.8157 | 0.6559 | 0.9766 | 0.0843 | 20 | 7e-04 |
bLength | -0.3527 | -0.5164 | -0.1847 | 0.0836 | 47 | 7e-04 |
Convergence | Iterations |
---|---|
1 | 1000 |
Site Fidelity - Wp
Parameter | Estimate | Lower | Upper | SD | Error | Significance |
---|---|---|---|---|---|---|
bFidelity | 0.7131 | 0.4206 | 1.0016 | 0.1474 | 41 | 0.0007 |
bLength | -0.1098 | -0.3932 | 0.1607 | 0.1463 | 250 | 0.4680 |
Convergence | Iterations |
---|---|
1 | 1000 |
Capture Efficiency - Mountain Whitefish - Subadult
Parameter | Estimate | Lower | Upper | SD | Error | Significance |
---|---|---|---|---|---|---|
bEfficiency | -4.8665 | -5.3606 | -4.494 | 0.2196 | 9 | 0.001 |
sEfficiencySessionYear | 0.4380 | 0.0560 | 1.072 | 0.2620 | 120 | 0.001 |
Convergence | Iterations |
---|---|
1.02 | 10000 |
Capture Efficiency - Mountain Whitefish - Adult
Parameter | Estimate | Lower | Upper | SD | Error | Significance |
---|---|---|---|---|---|---|
bEfficiency | -5.2437 | -5.6100 | -4.9354 | 0.1689 | 6 | 0.001 |
sEfficiencySessionYear | 0.3254 | 0.0575 | 0.7609 | 0.1850 | 110 | 0.001 |
Convergence | Iterations |
---|---|
1.02 | 10000 |
Capture Efficiency - Rainbow Trout - Subadult
Parameter | Estimate | Lower | Upper | SD | Error | Significance |
---|---|---|---|---|---|---|
bEfficiency | -3.3330 | -3.4698 | -3.2029 | 0.0677 | 4 | 0.001 |
sEfficiencySessionYear | 0.3769 | 0.2572 | 0.5232 | 0.0664 | 35 | 0.001 |
Convergence | Iterations |
---|---|
1.01 | 10000 |
Capture Efficiency - Rainbow Trout - Adult
Parameter | Estimate | Lower | Upper | SD | Error | Significance |
---|---|---|---|---|---|---|
bEfficiency | -4.0469 | -4.2146 | -3.8918 | 0.0806 | 4 | 0.001 |
sEfficiencySessionYear | 0.2415 | 0.0418 | 0.4559 | 0.1130 | 86 | 0.001 |
Convergence | Iterations |
---|---|
1.02 | 20000 |
Capture Efficiency - Walleye - Adult
Parameter | Estimate | Lower | Upper | SD | Error | Significance |
---|---|---|---|---|---|---|
bEfficiency | -4.4552 | -4.7121 | -4.2124 | 0.1278 | 6 | 0.001 |
sEfficiencySessionYear | 0.6317 | 0.4017 | 0.9357 | 0.1328 | 42 | 0.001 |
Convergence | Iterations |
---|---|
1.01 | 10000 |
Abundance - Mountain Whitefish - Subadult
Parameter | Estimate | Lower | Upper | SD | Error | Significance |
---|---|---|---|---|---|---|
bDensity | 5.23050 | 4.80530 | 5.67640 | 0.22090 | 8 | 0.001 |
bVisitType[1] | 1.00000 | 1.00000 | 1.00000 | 0.00000 | 0 | 0.001 |
bVisitType[2] | 3.52400 | 2.89800 | 4.27300 | 0.34800 | 20 | 0.001 |
sDensitySite | 0.79980 | 0.64880 | 0.99690 | 0.09140 | 22 | 0.001 |
sDensitySiteYear | 0.45780 | 0.39060 | 0.52320 | 0.03440 | 14 | 0.001 |
sDensityYear | 0.79200 | 0.53850 | 1.21870 | 0.17270 | 43 | 0.001 |
sDispersion | 0.51317 | 0.46829 | 0.55429 | 0.02255 | 8 | 0.001 |
Convergence | Iterations |
---|---|
1.02 | 80000 |
Abundance - Mountain Whitefish - Adult
Parameter | Estimate | Lower | Upper | SD | Error | Significance |
---|---|---|---|---|---|---|
bDensity | 6.51500 | 6.14650 | 6.87570 | 0.19280 | 6 | 0.001 |
bVisitType[1] | 1.00000 | 1.00000 | 1.00000 | 0.00000 | 0 | 0.001 |
bVisitType[2] | 4.29600 | 3.53000 | 5.26100 | 0.44100 | 20 | 0.001 |
sDensitySite | 1.12070 | 0.91680 | 1.36950 | 0.11710 | 20 | 0.001 |
sDensitySiteYear | 0.43110 | 0.37410 | 0.49460 | 0.03080 | 14 | 0.001 |
sDensityYear | 0.40810 | 0.25760 | 0.68160 | 0.10500 | 52 | 0.001 |
sDispersion | 0.53859 | 0.50496 | 0.57326 | 0.01785 | 6 | 0.001 |
Convergence | Iterations |
---|---|
1.08 | 40000 |
Abundance - Rainbow Trout - Subadult
Parameter | Estimate | Lower | Upper | SD | Error | Significance |
---|---|---|---|---|---|---|
bDensity | 4.86340 | 4.54000 | 5.17690 | 0.16720 | 7 | 0.001 |
bVisitType[1] | 1.00000 | 1.00000 | 1.00000 | 0.00000 | 0 | 0.001 |
bVisitType[2] | 3.64400 | 3.13600 | 4.22100 | 0.28200 | 15 | 0.001 |
sDensitySite | 0.77470 | 0.63960 | 0.94180 | 0.07850 | 20 | 0.001 |
sDensitySiteYear | 0.34730 | 0.29690 | 0.39990 | 0.02630 | 15 | 0.001 |
sDensityYear | 0.34860 | 0.22740 | 0.56640 | 0.08670 | 49 | 0.001 |
sDispersion | 0.39992 | 0.36967 | 0.42961 | 0.01537 | 7 | 0.001 |
Convergence | Iterations |
---|---|
1.05 | 10000 |
Abundance - Rainbow Trout - Adult
Parameter | Estimate | Lower | Upper | SD | Error | Significance |
---|---|---|---|---|---|---|
bDensity | 5.30300 | 5.0923 | 5.50690 | 0.10180 | 4 | 0.001 |
bVisitType[1] | 1.00000 | 1.0000 | 1.00000 | 0.00000 | 0 | 0.001 |
bVisitType[2] | 3.60200 | 3.1070 | 4.17700 | 0.27400 | 15 | 0.001 |
sDensitySite | 0.69250 | 0.5624 | 0.84230 | 0.07060 | 20 | 0.001 |
sDensitySiteYear | 0.26290 | 0.2061 | 0.31940 | 0.02840 | 22 | 0.001 |
sDensityYear | 0.18570 | 0.1103 | 0.30540 | 0.05190 | 53 | 0.001 |
sDispersion | 0.39761 | 0.3638 | 0.43281 | 0.01774 | 9 | 0.001 |
Convergence | Iterations |
---|---|
1.03 | 10000 |
Abundance - Walleye - Adult
Parameter | Estimate | Lower | Upper | SD | Error | Significance |
---|---|---|---|---|---|---|
bDensity | 5.42750 | 5.17610 | 5.71860 | 0.1321 | 5 | 0.001 |
bVisitType[1] | 1.00000 | 1.00000 | 1.00000 | 0.0000 | 0 | 0.001 |
bVisitType[2] | 3.64200 | 3.03700 | 4.35300 | 0.3400 | 18 | 0.001 |
sDensitySite | 0.38880 | 0.28380 | 0.51710 | 0.0597 | 30 | 0.001 |
sDensitySiteYear | 0.21960 | 0.16680 | 0.26910 | 0.0263 | 23 | 0.001 |
sDensityYear | 0.49340 | 0.33800 | 0.75740 | 0.1077 | 43 | 0.001 |
sDispersion | 0.46791 | 0.43394 | 0.50448 | 0.0182 | 8 | 0.001 |
Convergence | Iterations |
---|---|
1.09 | 10000 |
Long-Term Trends
Parameter | Estimate | Lower | Upper | SD | Error | Significance |
---|---|---|---|---|---|---|
sTrend | 0.3652 | 0.1745 | 0.5719 | 0.1081 | 54 | 0.001 |
sValue | 0.8582 | 0.7778 | 0.9460 | 0.0422 | 10 | 0.001 |
Convergence | Iterations |
---|---|
1.07 | 10000 |
Figures
Condition
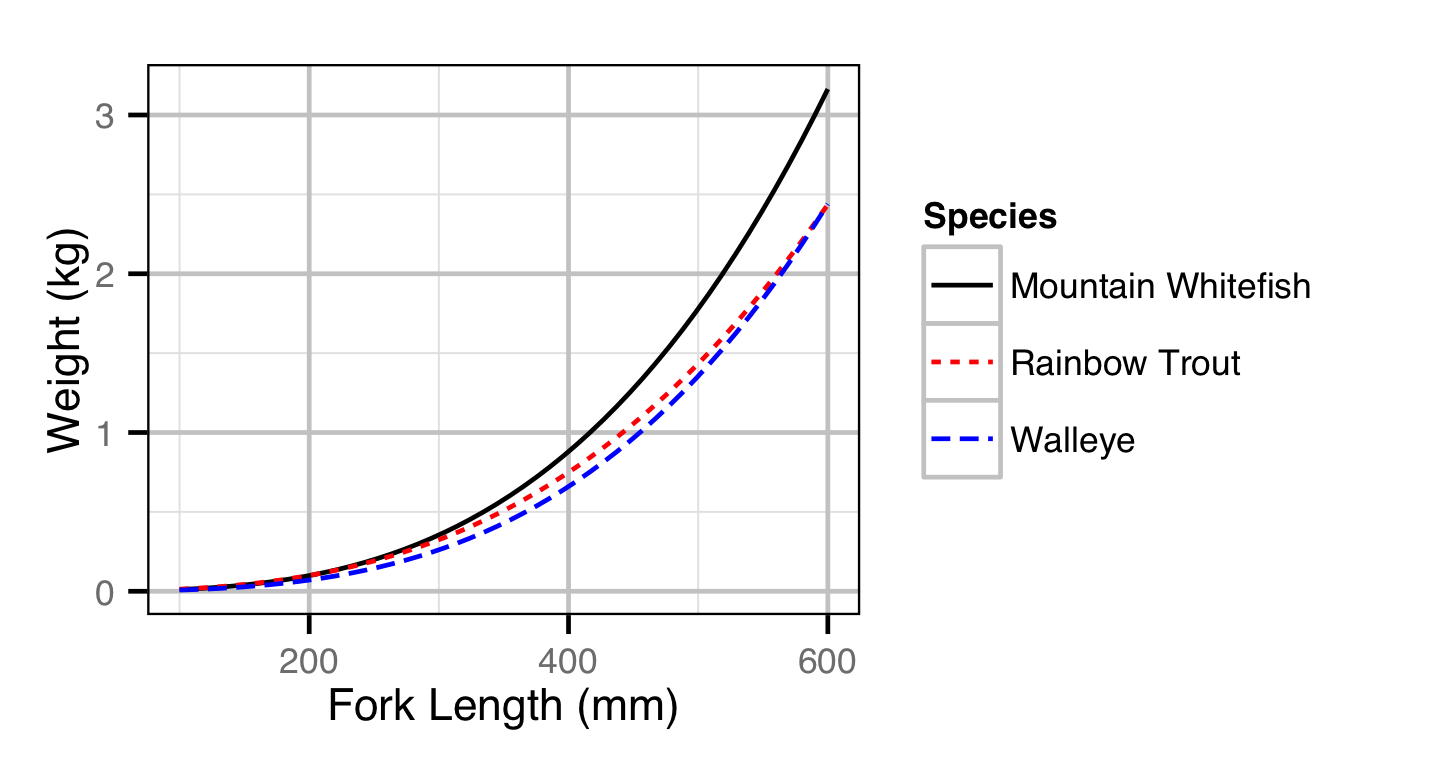
Condition - Mountain Whitefish - Subadult
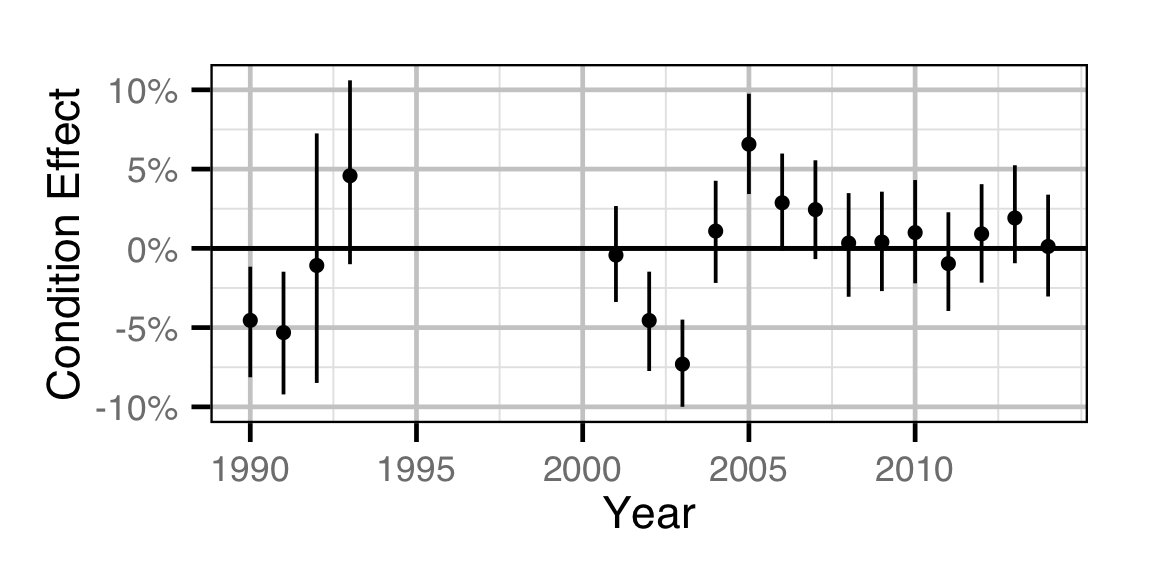
Condition - Mountain Whitefish - Adult
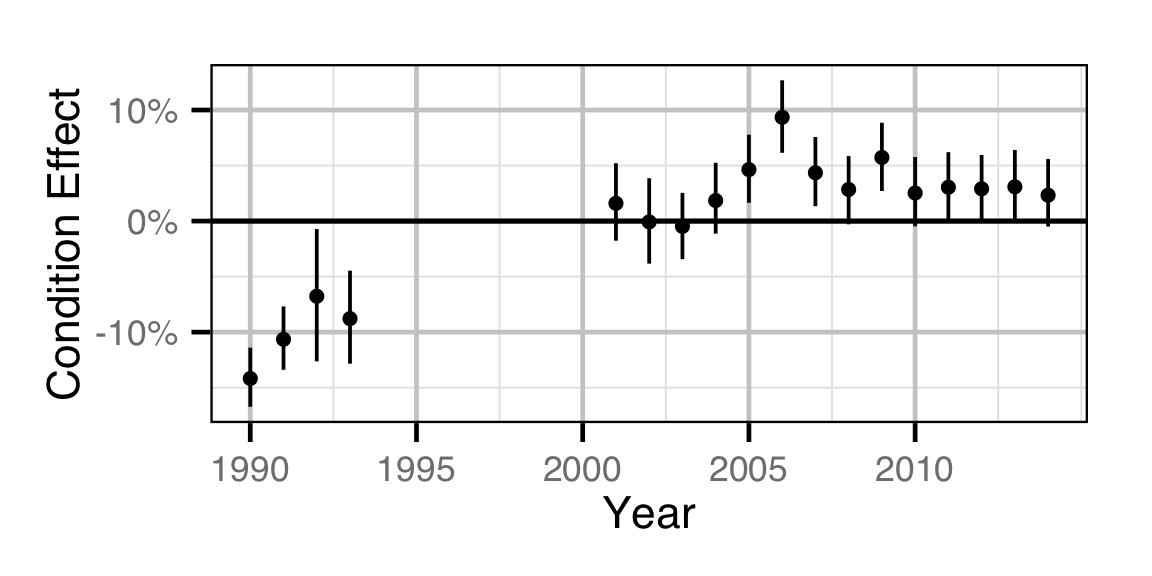
Condition - Rainbow Trout - Subadult
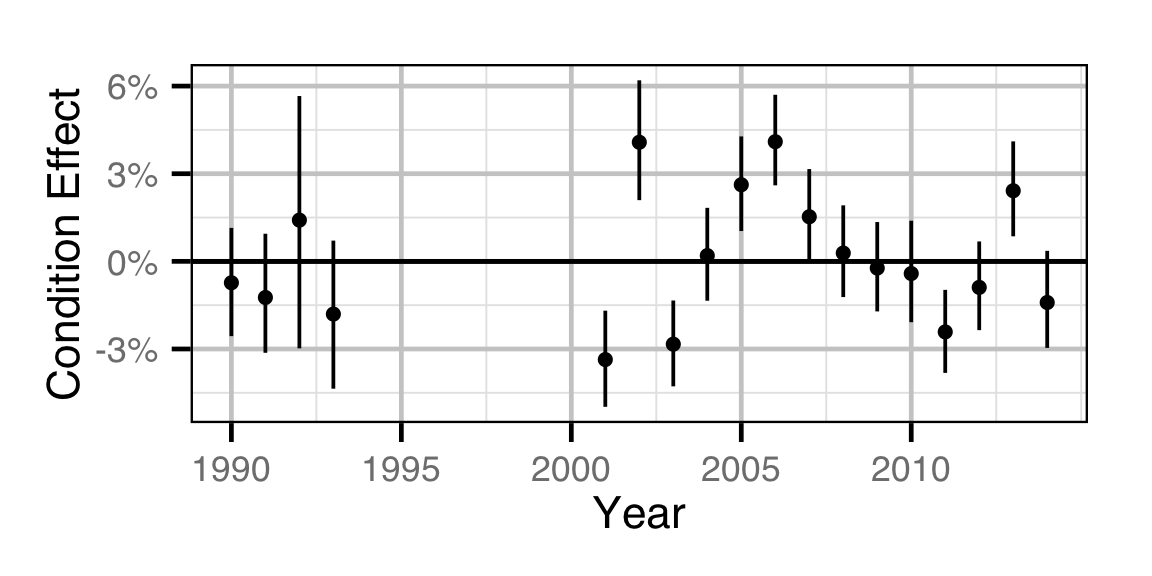
Condition - Rainbow Trout - Adult
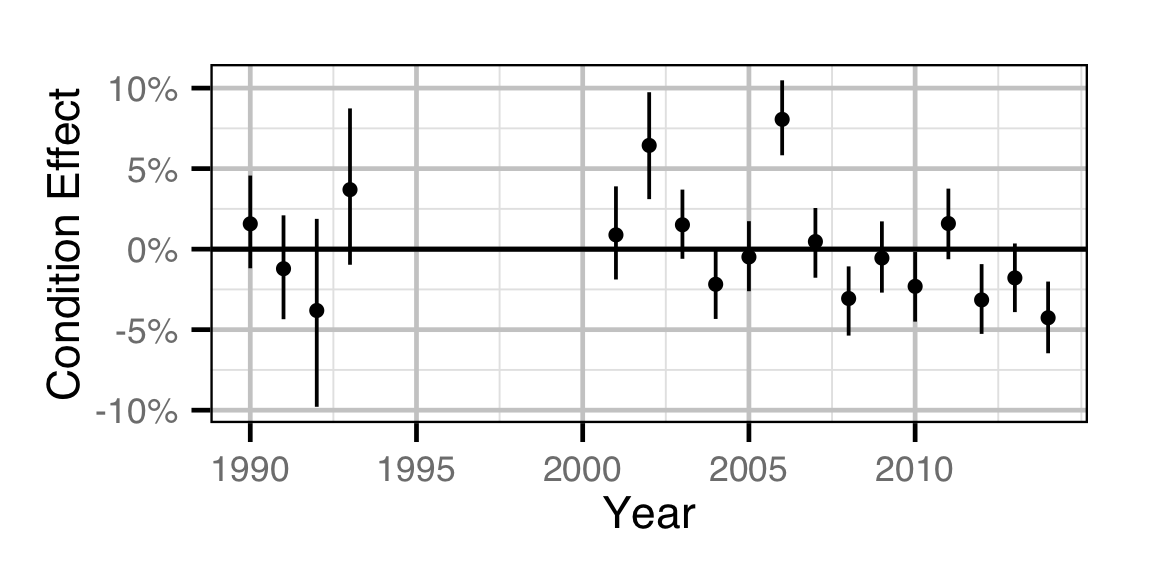
Condition - Walleye - Adult
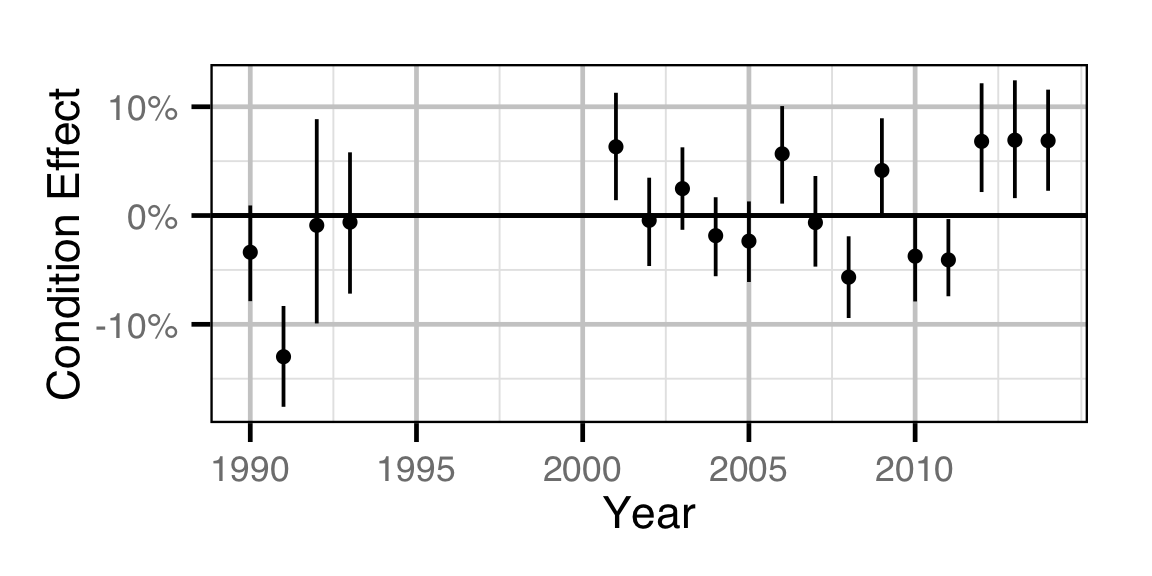
Growth
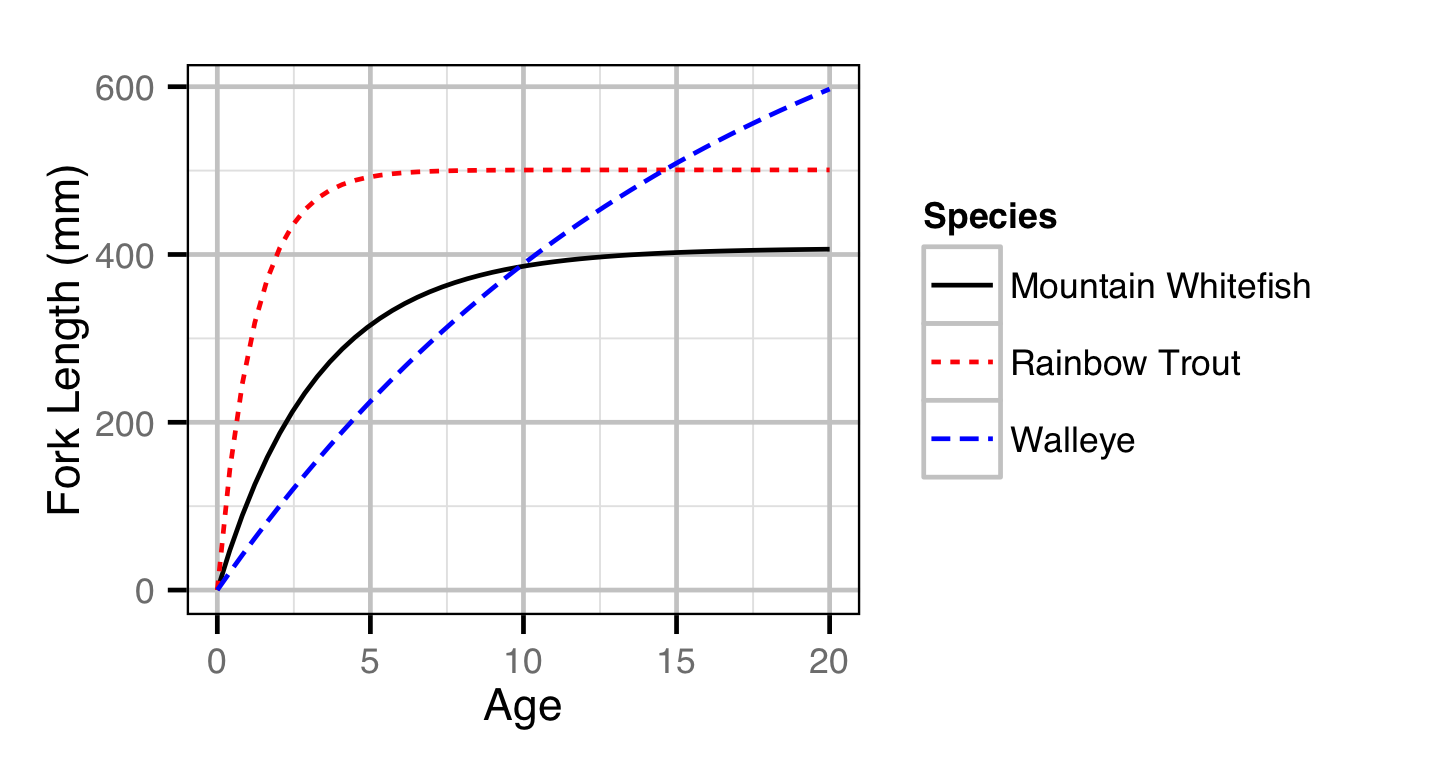
Growth - Mountain Whitefish
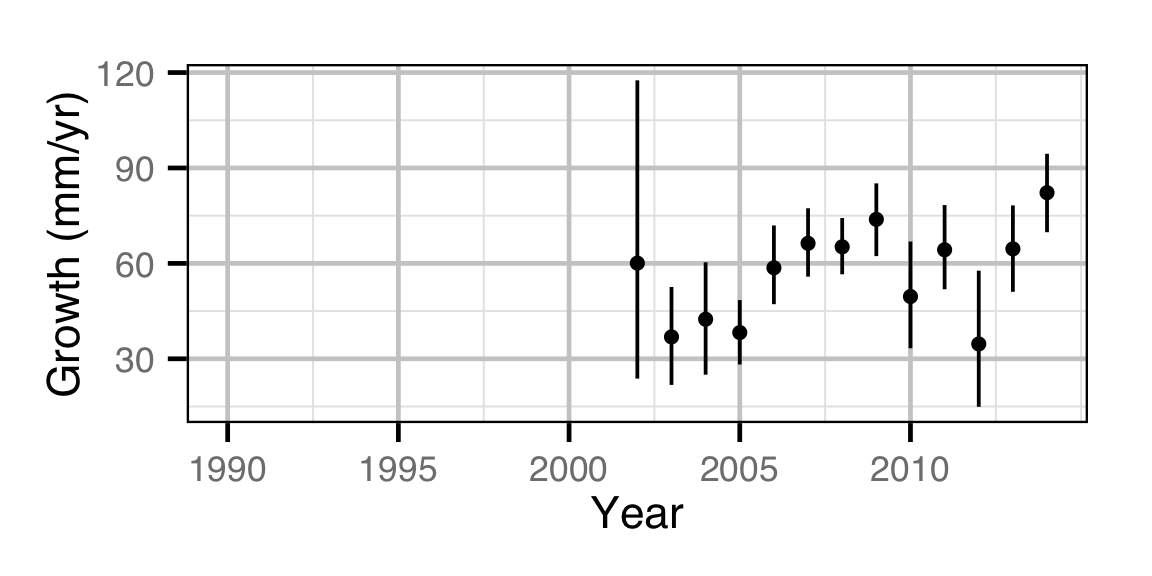
Growth - Rainbow Trout
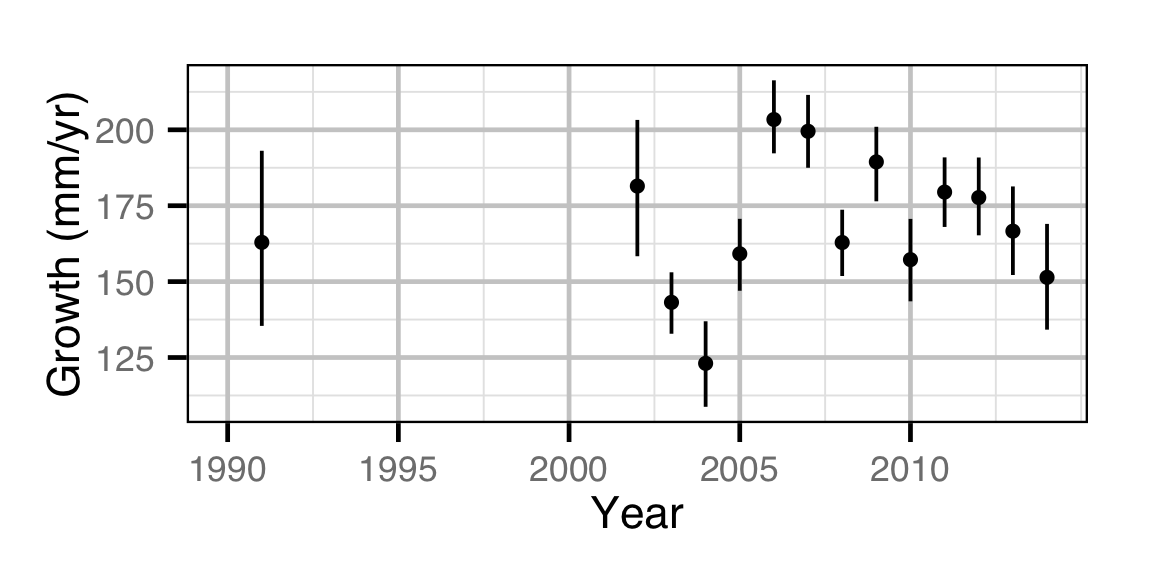
Growth - Walleye
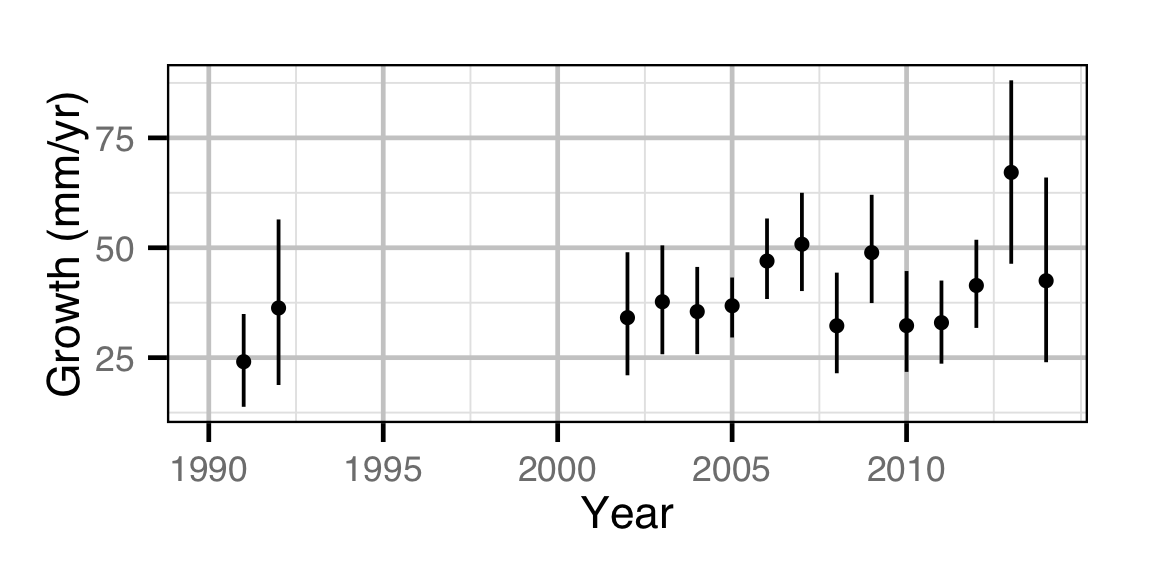
Length-At-Age - Mountain Whitefish
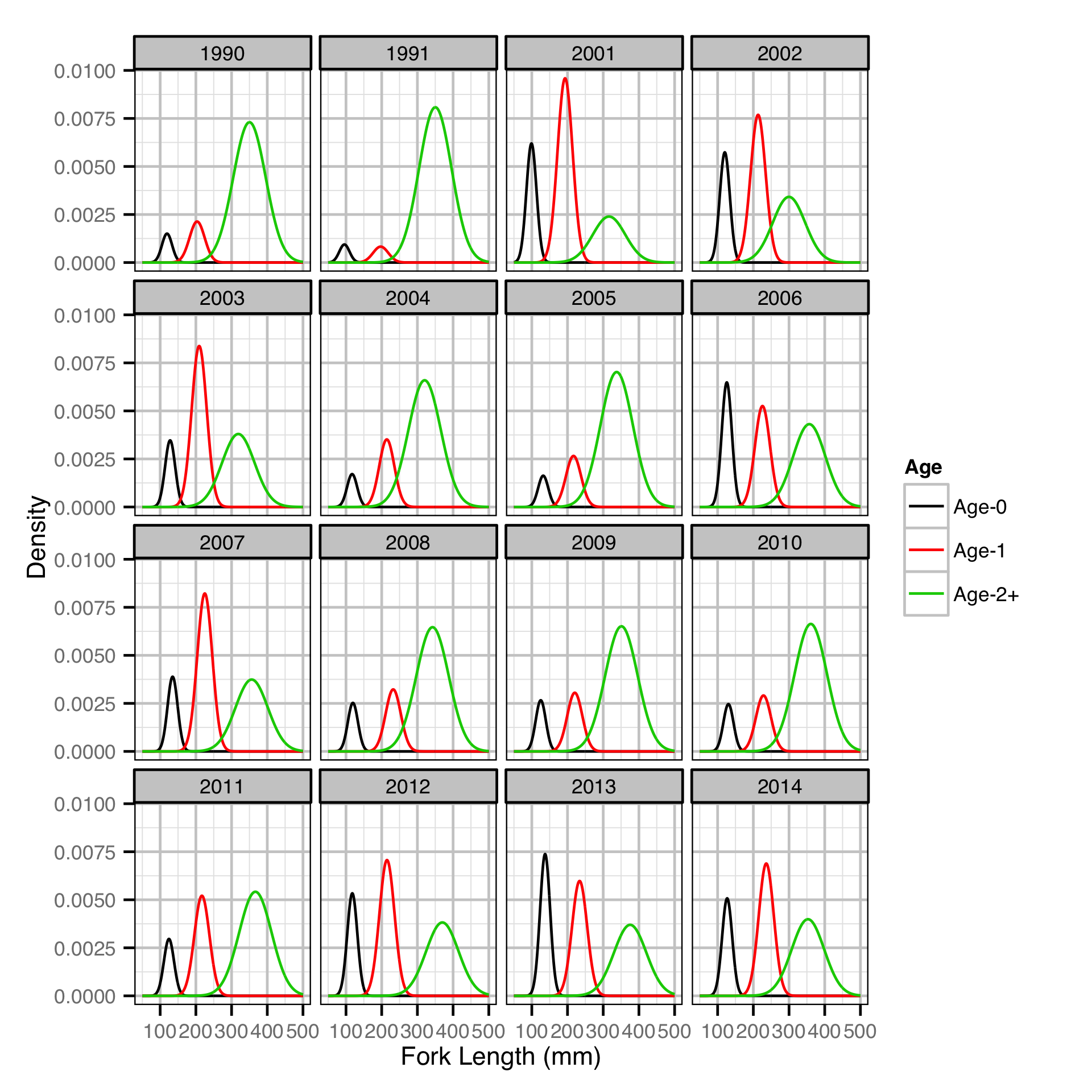
Length-At-Age - Mountain Whitefish - Age-0
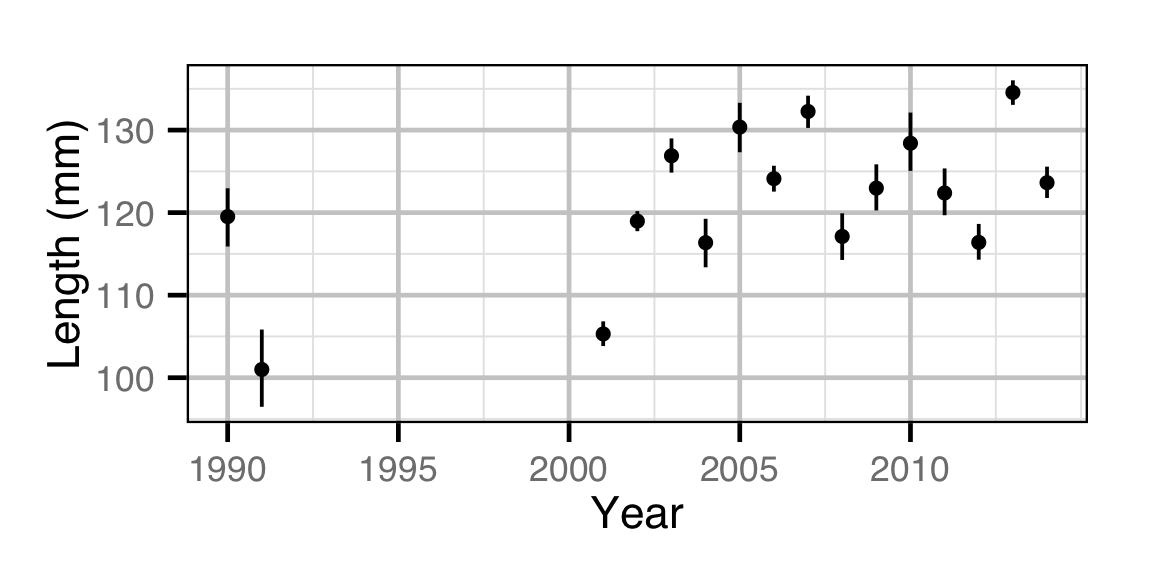
Length-At-Age - Mountain Whitefish - Age-1
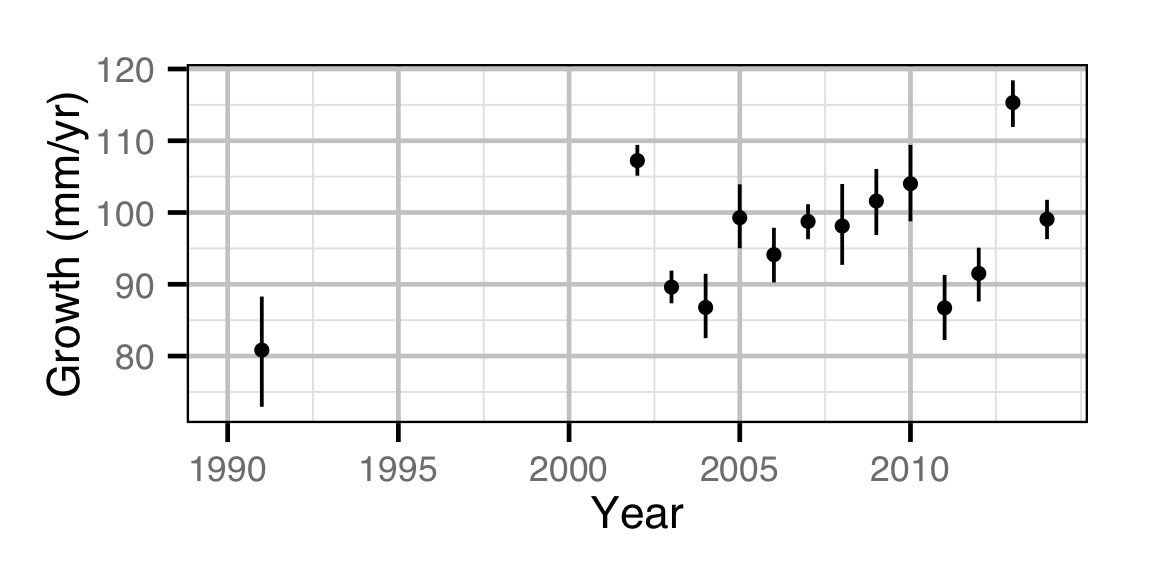
Length-At-Age - Rainbow Trout
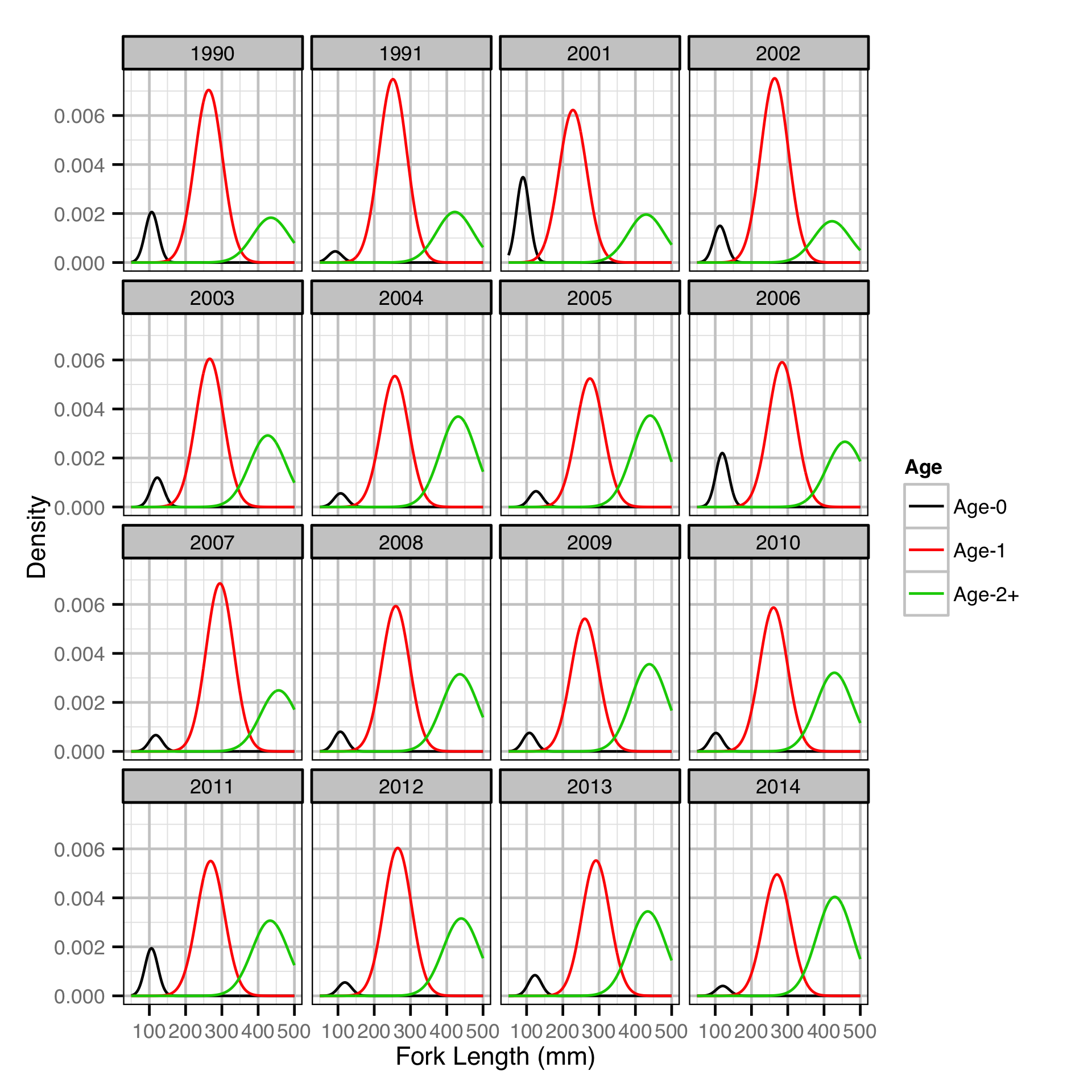
Length-At-Age - Rainbow Trout - Age-0
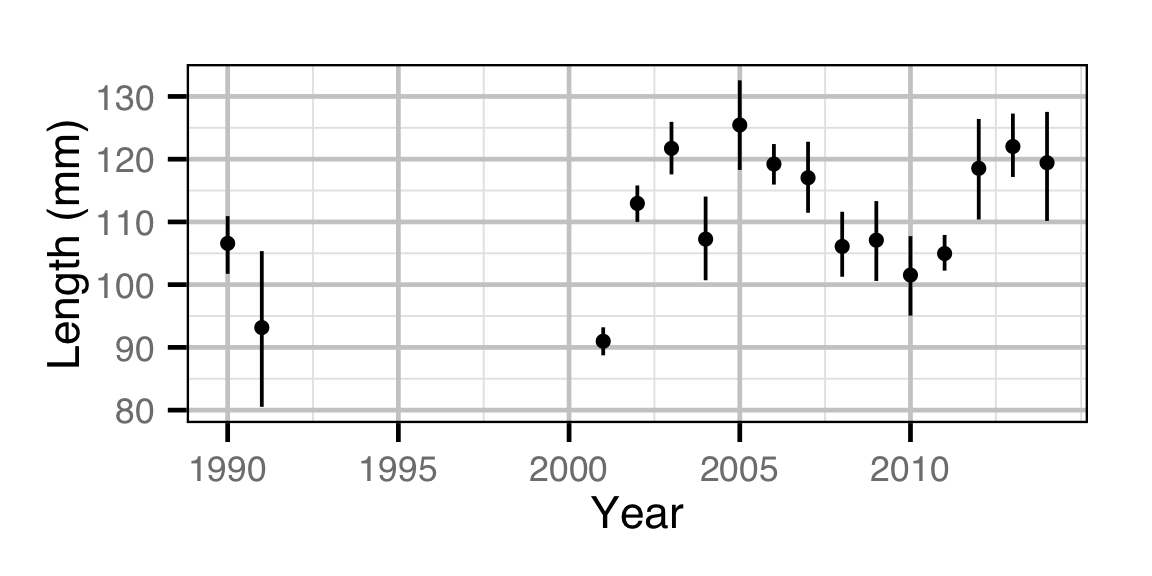
Length-At-Age - Rainbow Trout - Age-1
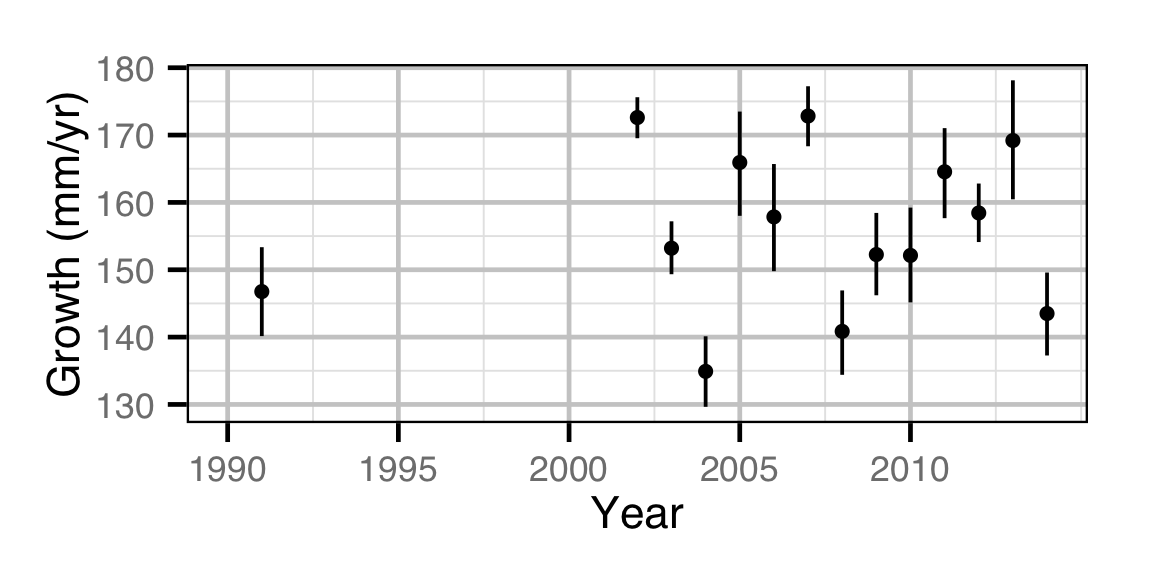
Observer Length Correction
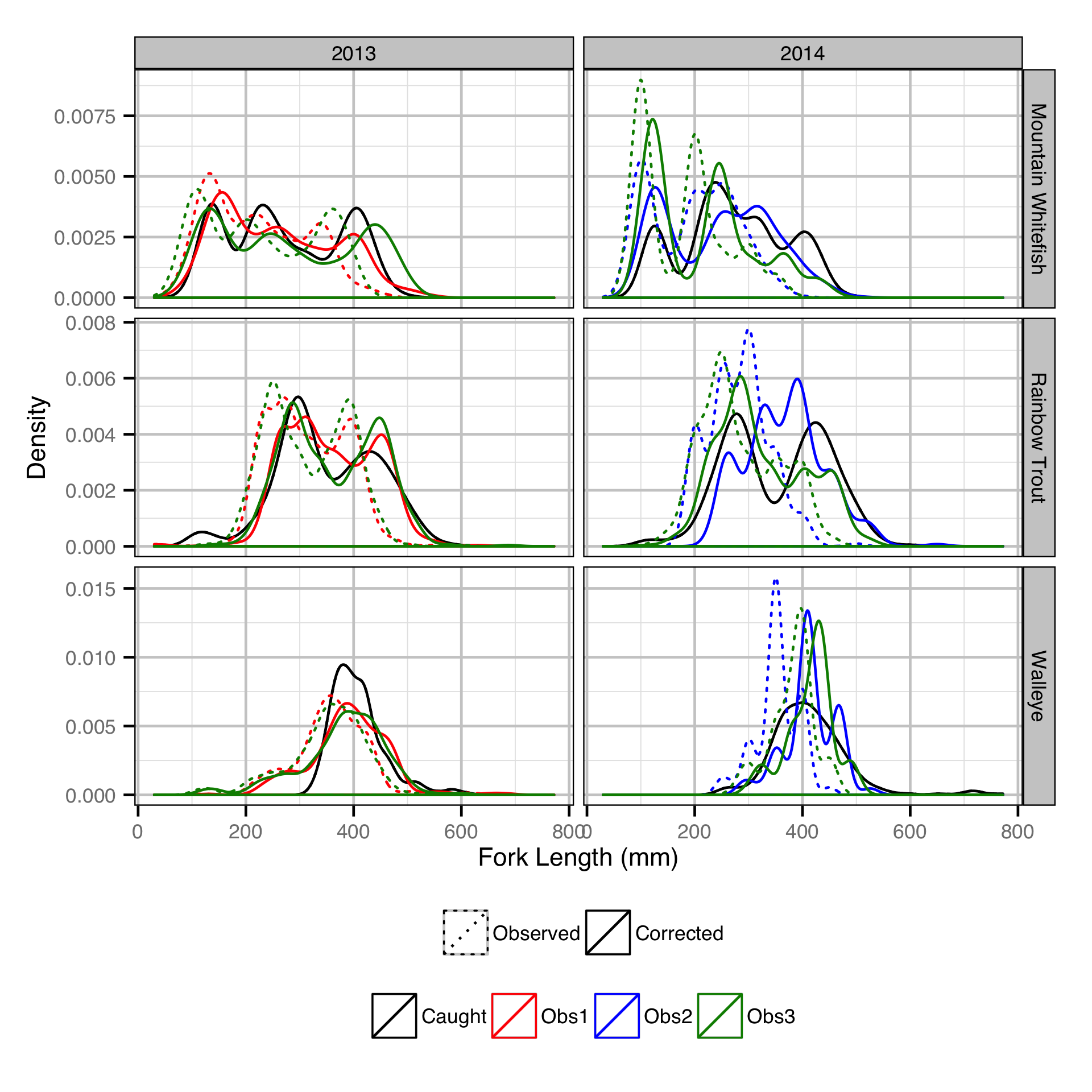
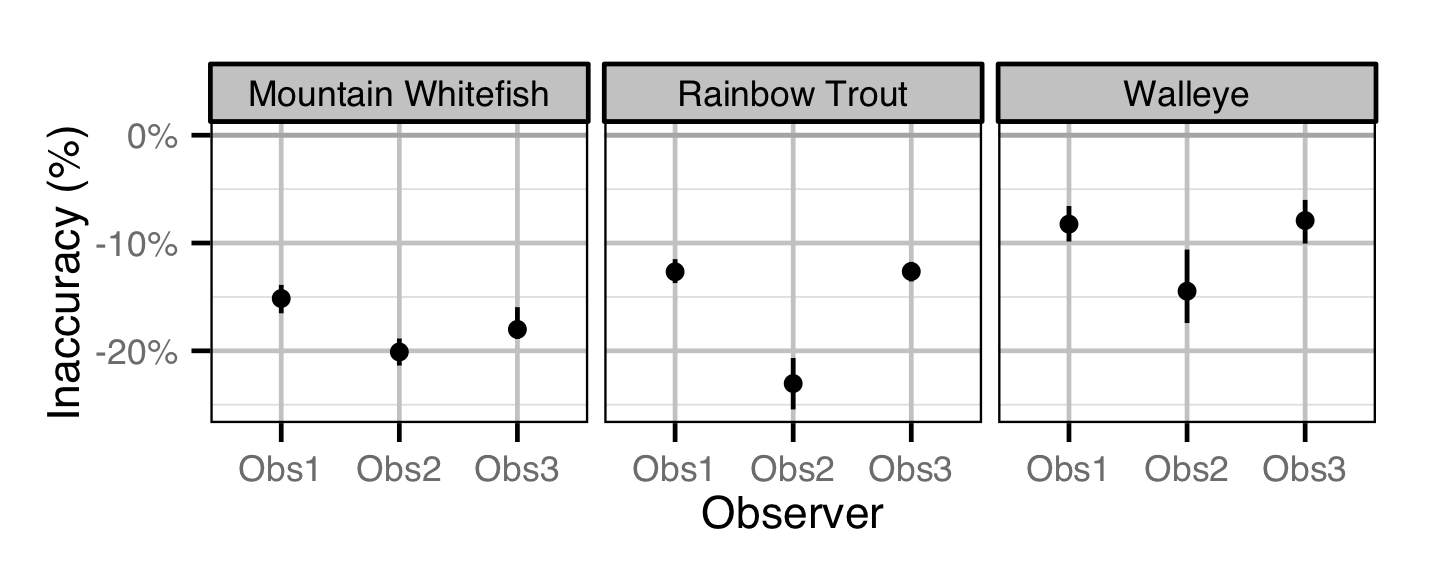
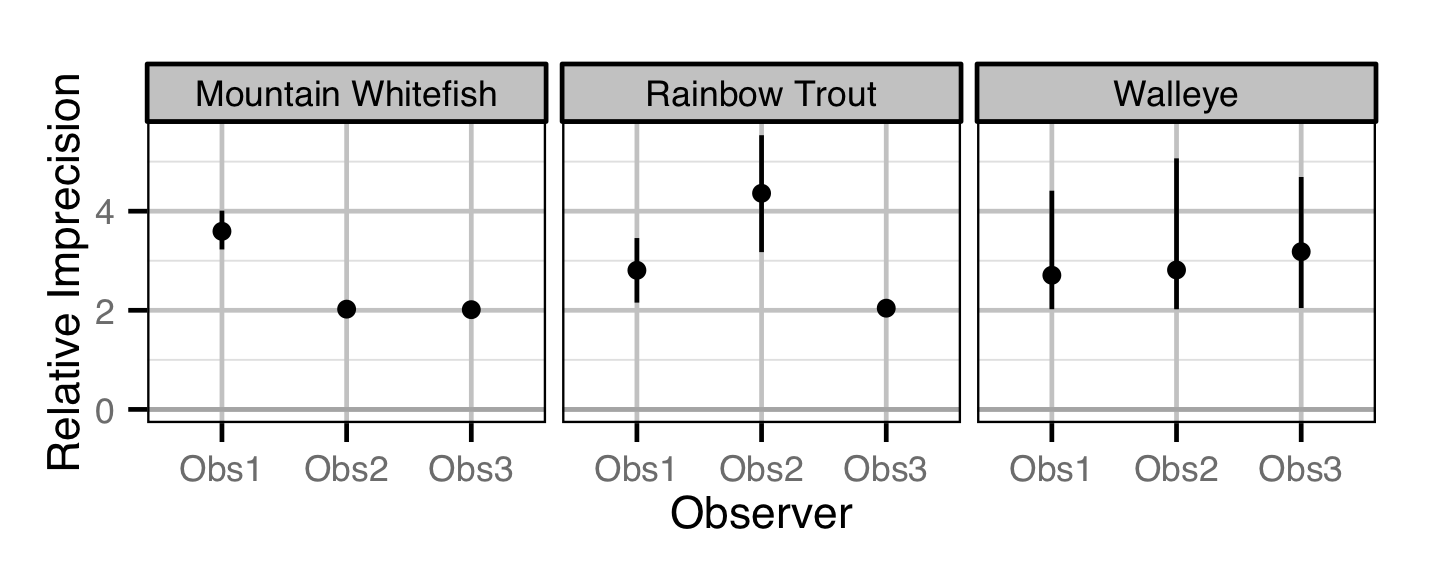
Survival - Mountain Whitefish - Subadult
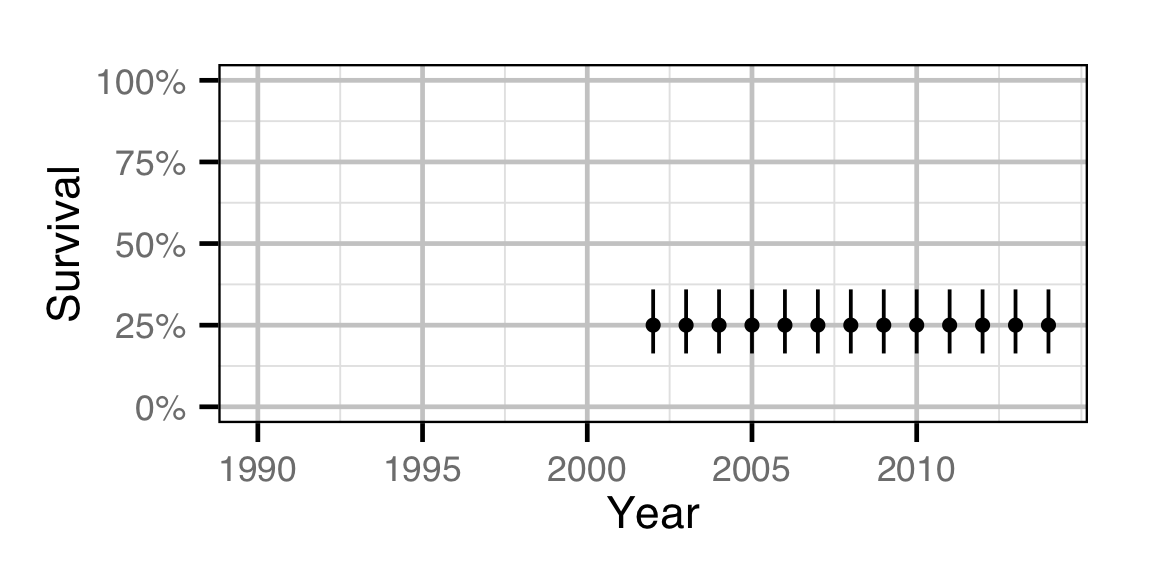
Survival - Mountain Whitefish - Adult
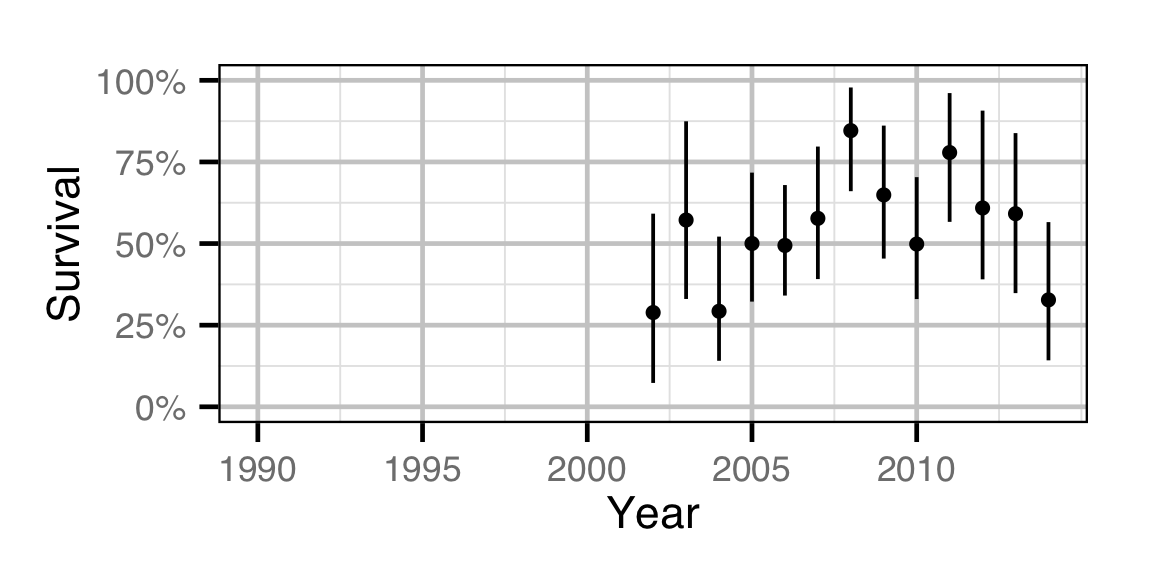
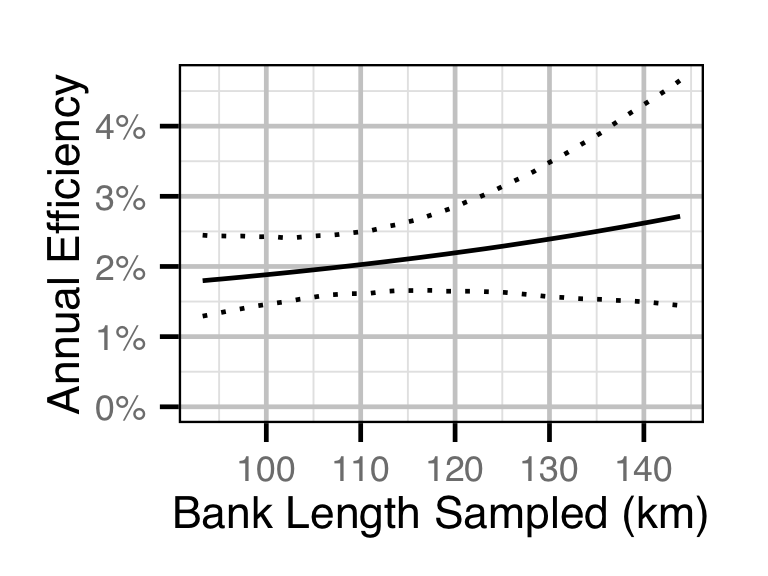
Survival - Rainbow Trout - Subadult
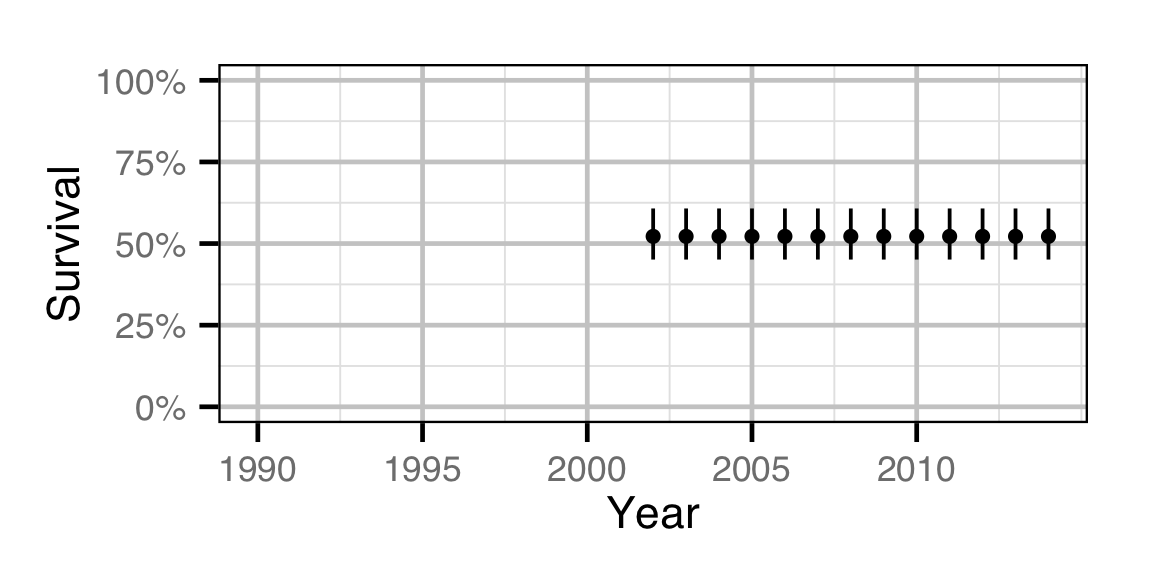
Survival - Rainbow Trout - Adult
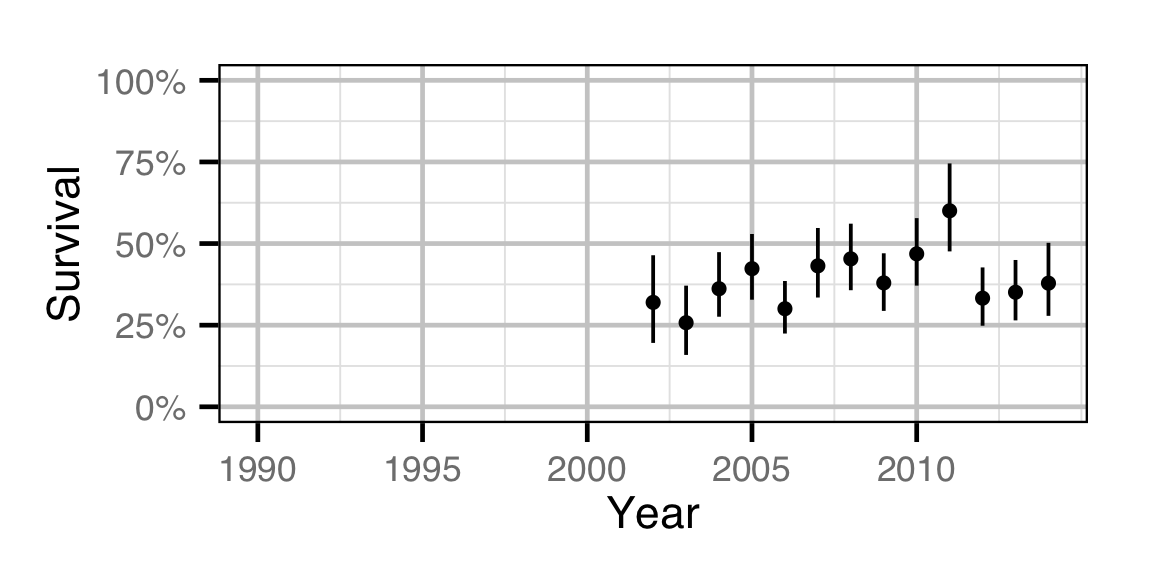
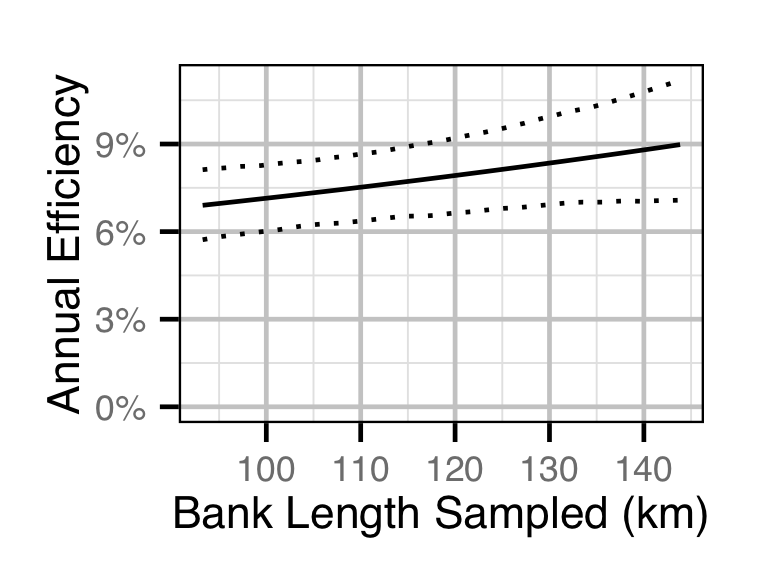
Survival - Walleye - Adult
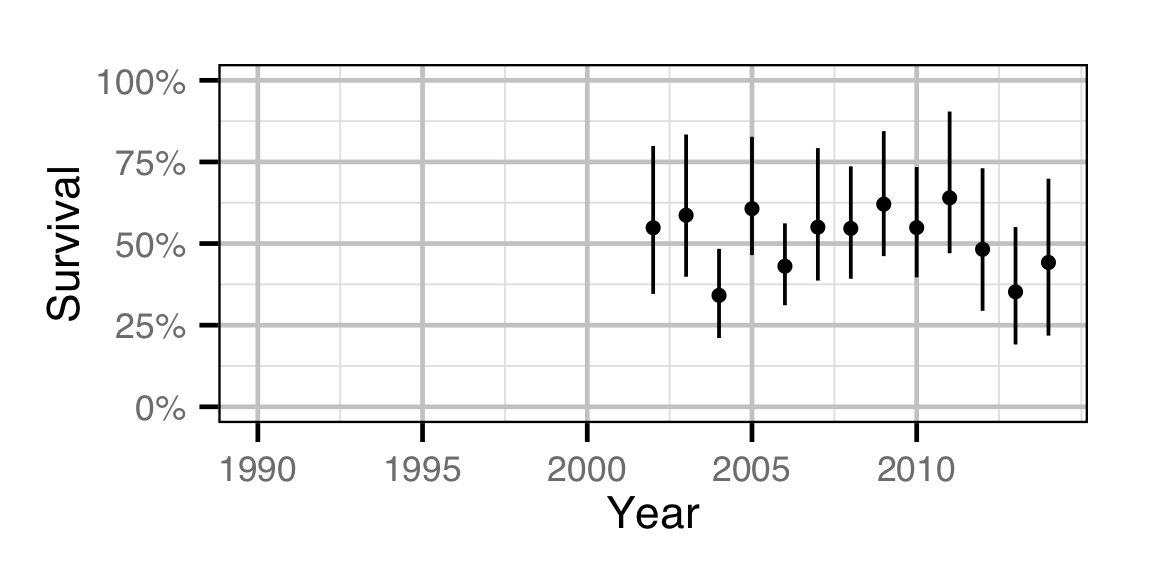
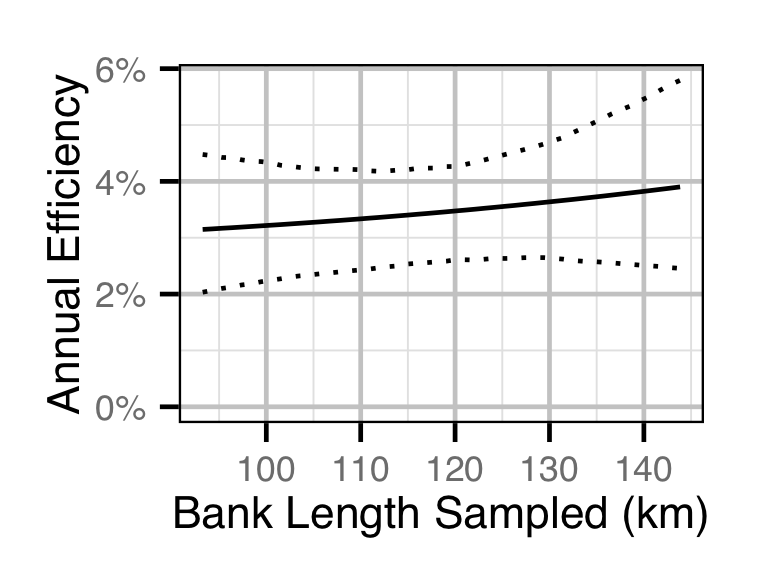
Site Fidelity
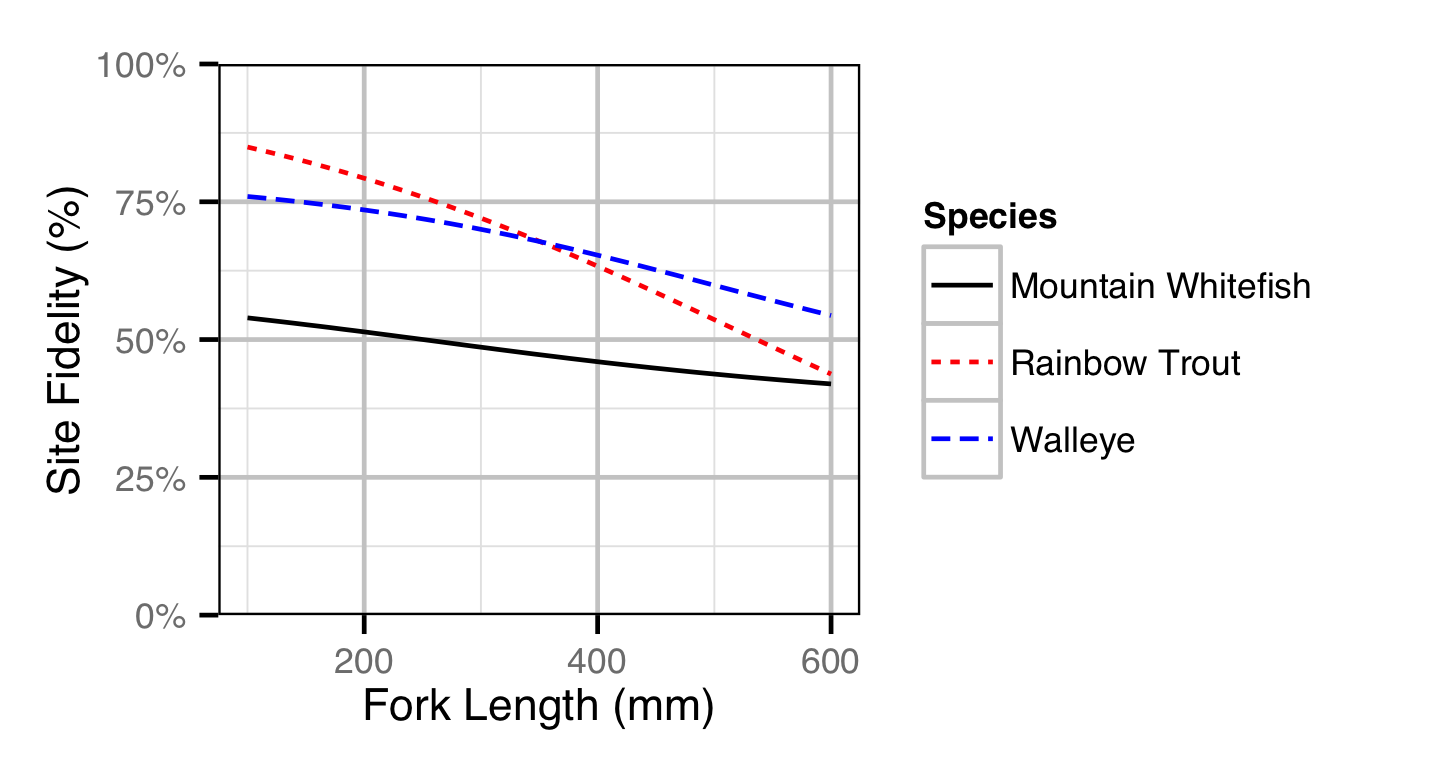
Capture Efficiency
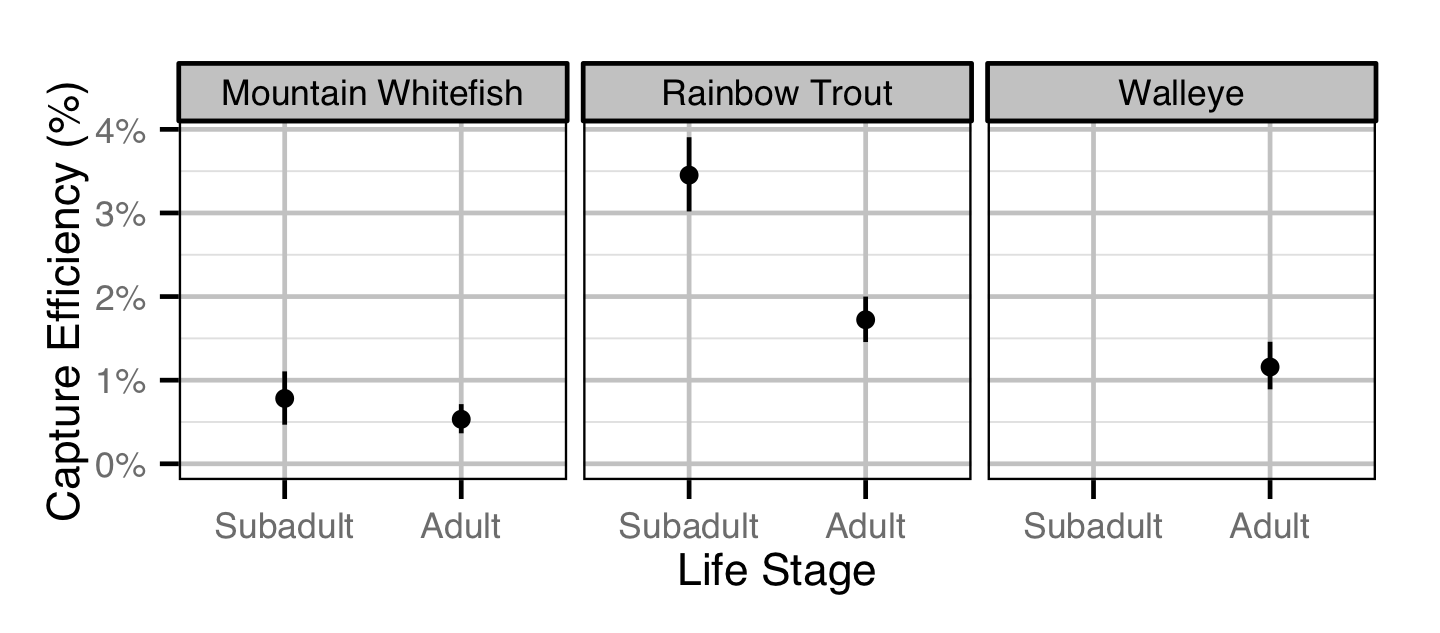
Capture Efficiency - Mountain Whitefish - Subadult
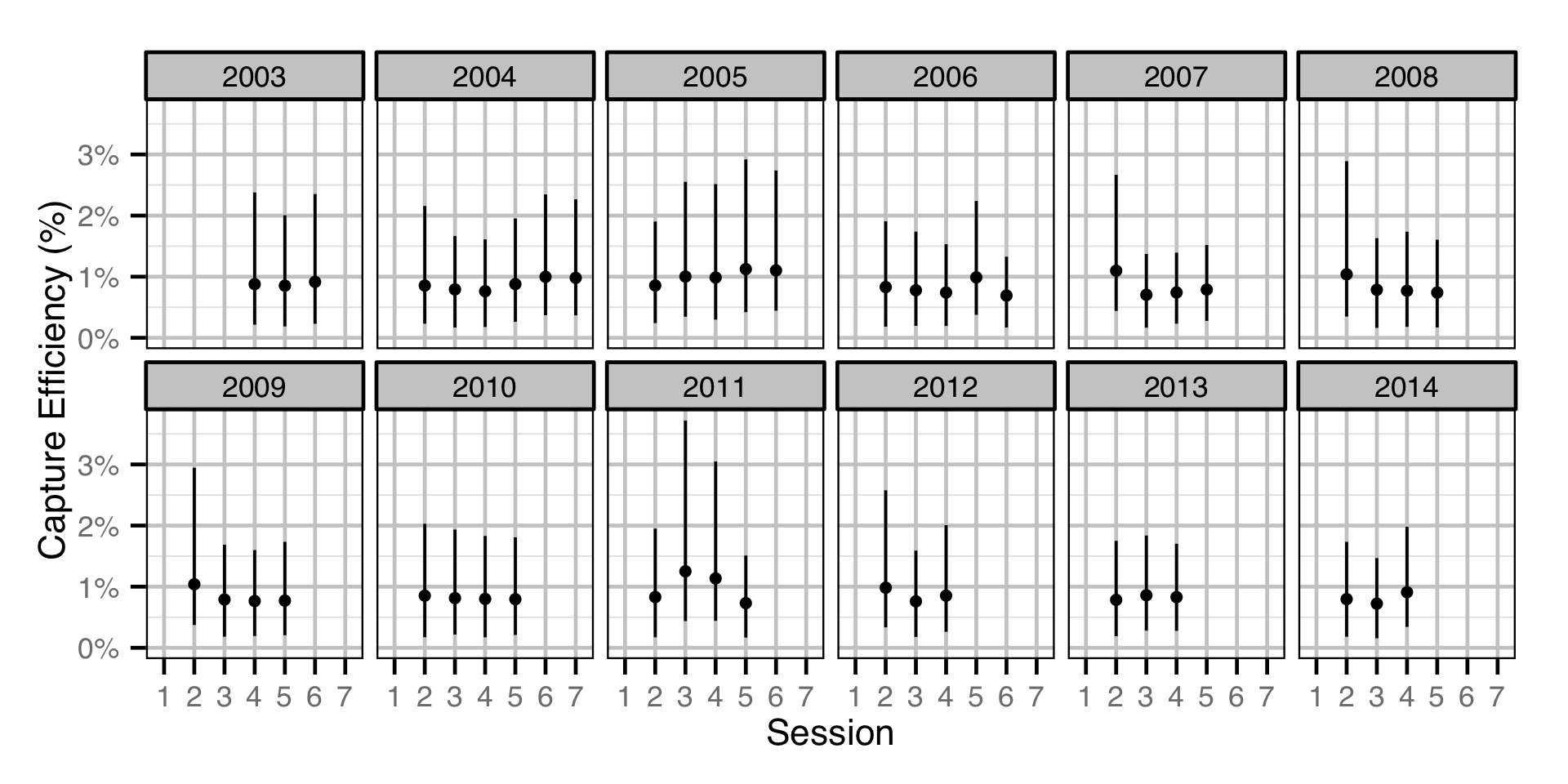
Capture Efficiency - Mountain Whitefish - Adult
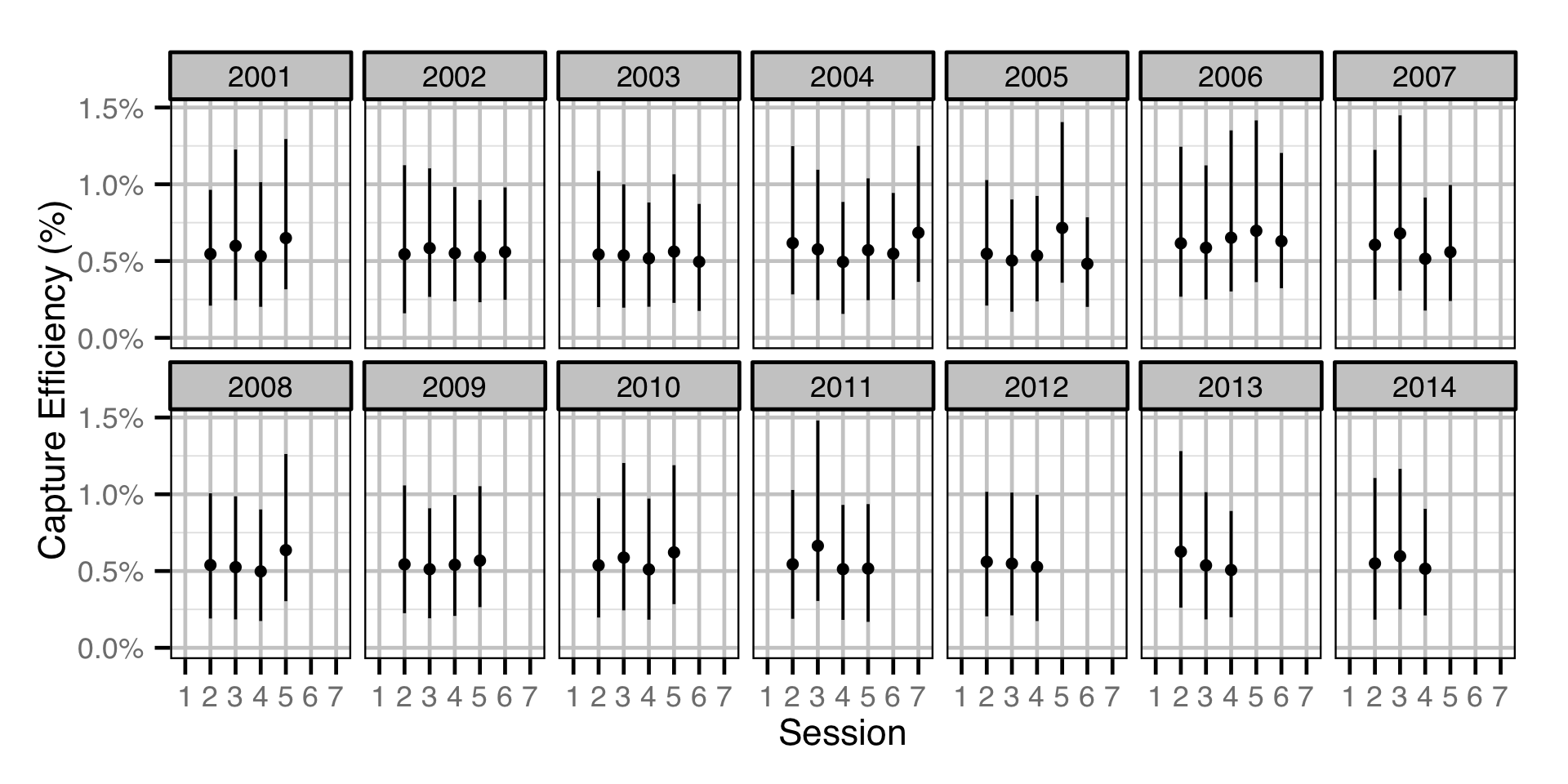
Capture Efficiency - Rainbow Trout - Subadult
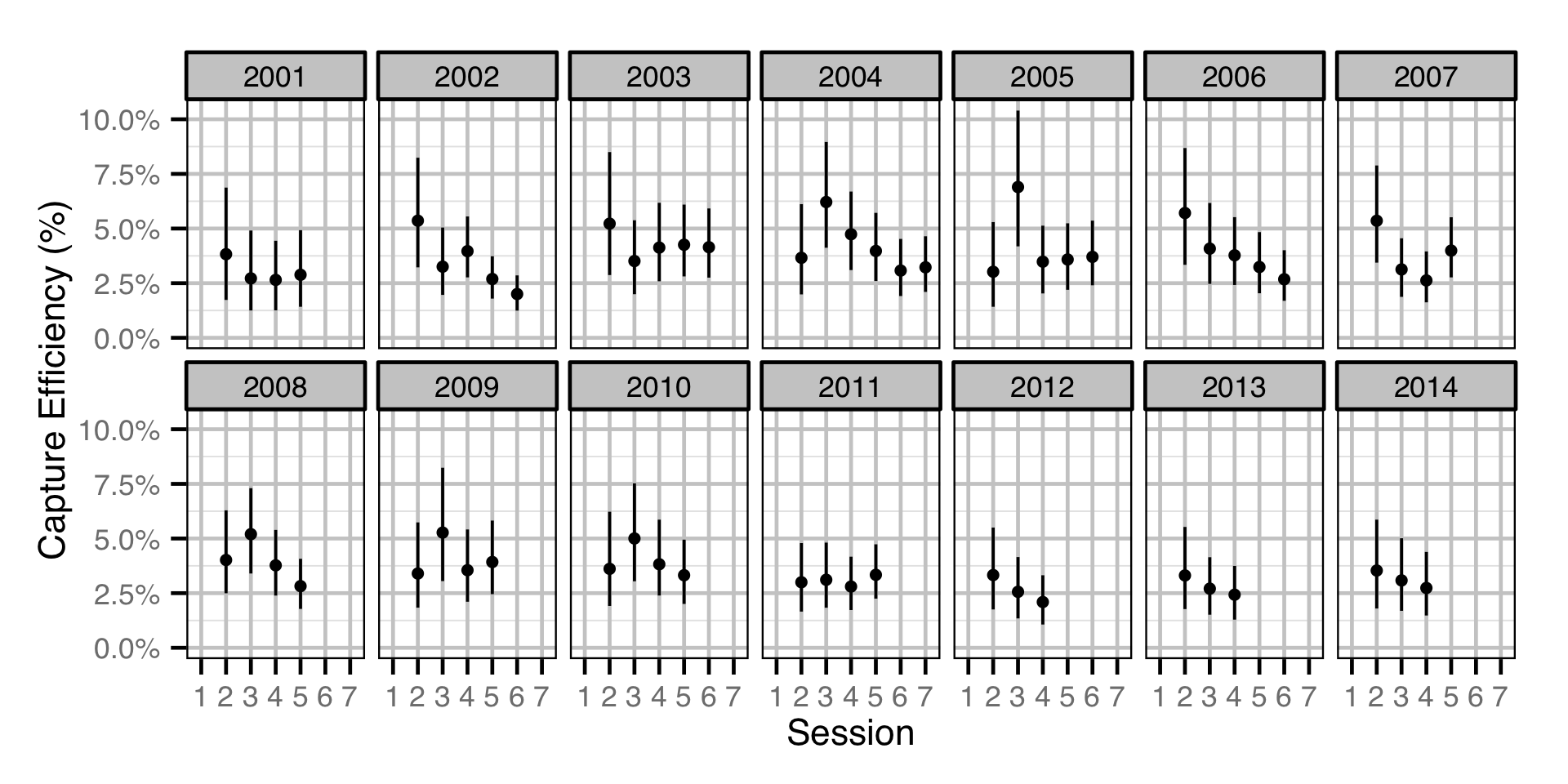
Capture Efficiency - Rainbow Trout - Adult
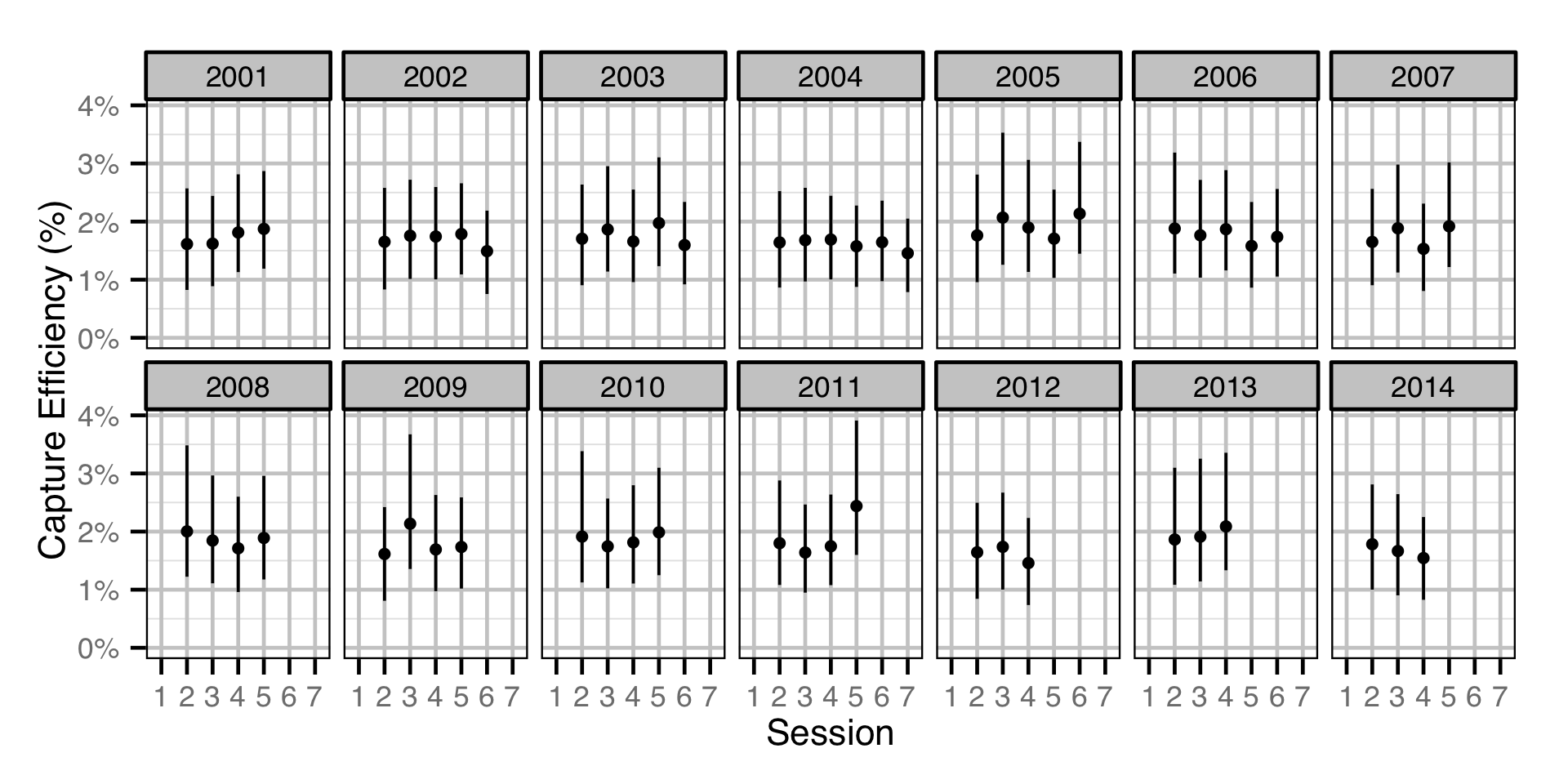
Capture Efficiency - Walleye - Adult
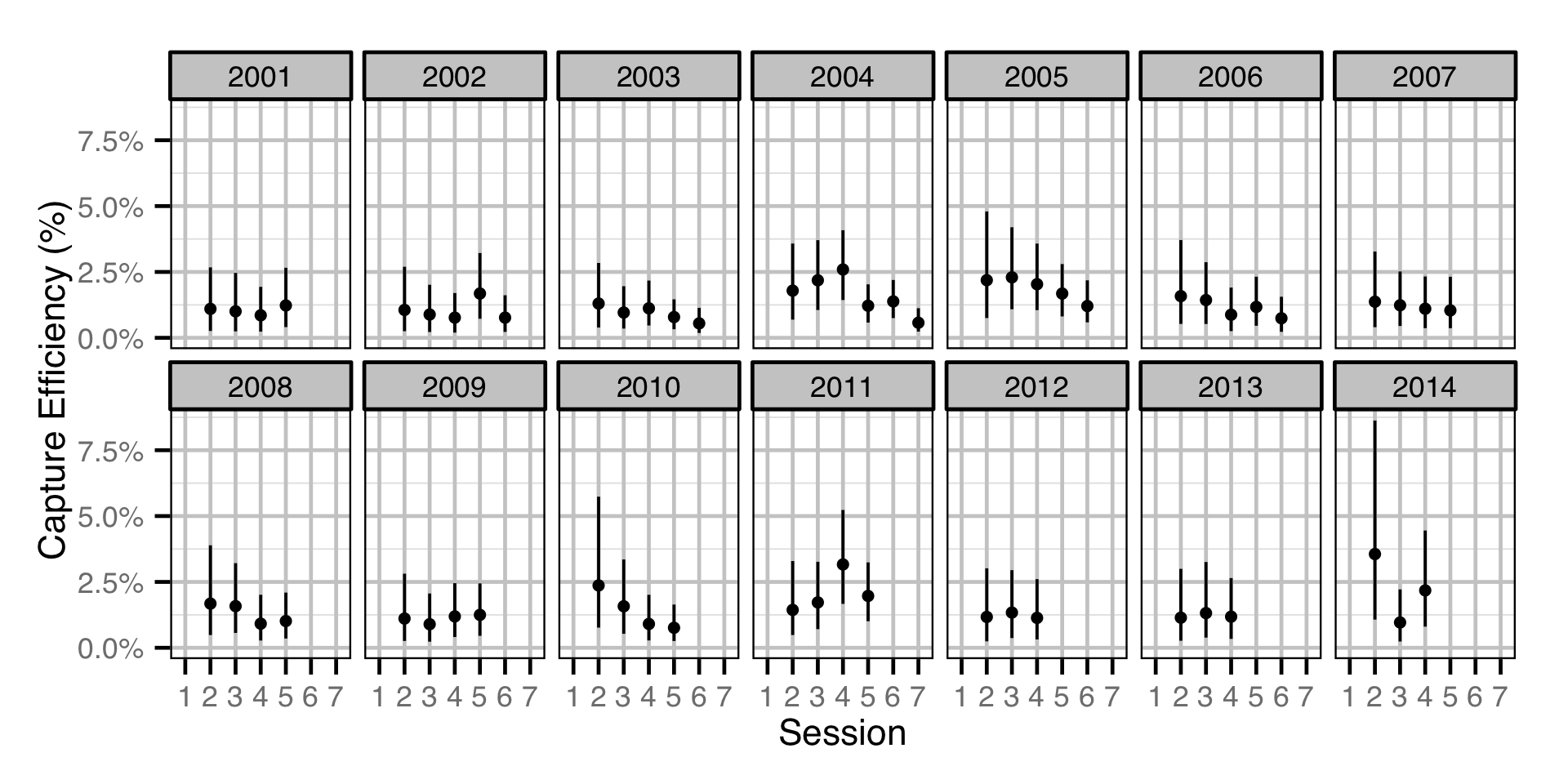
Abundance
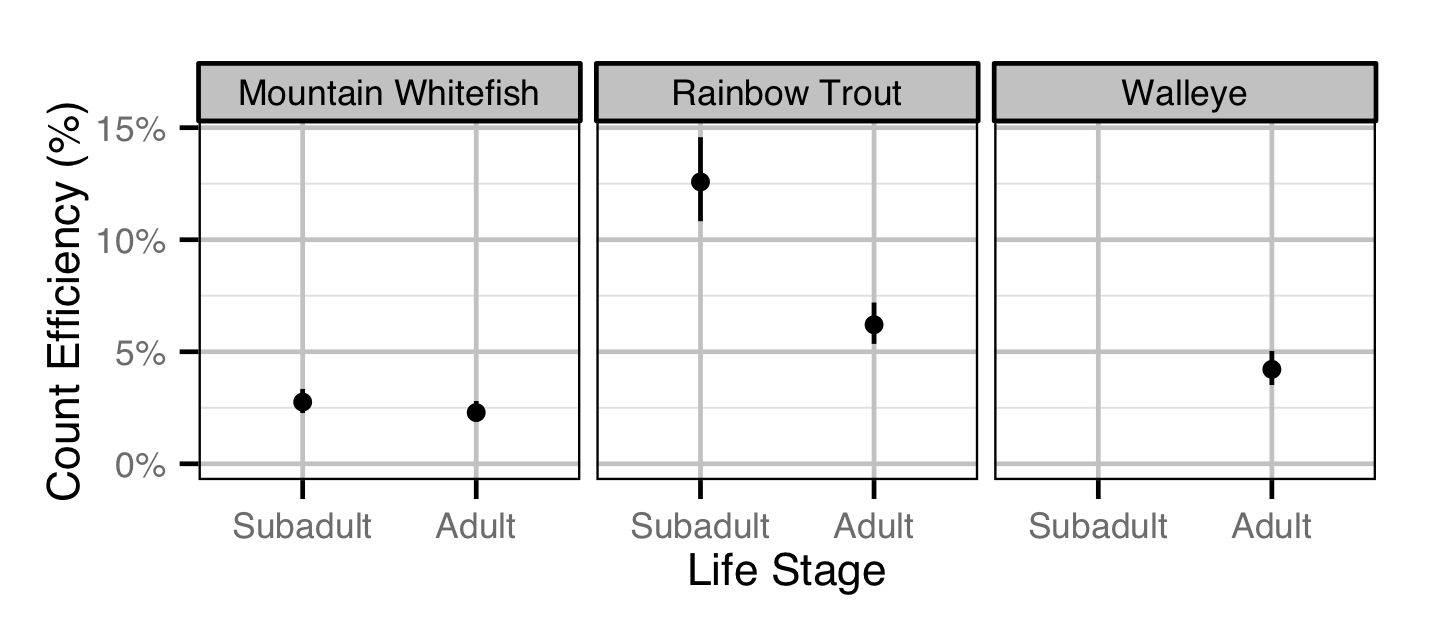
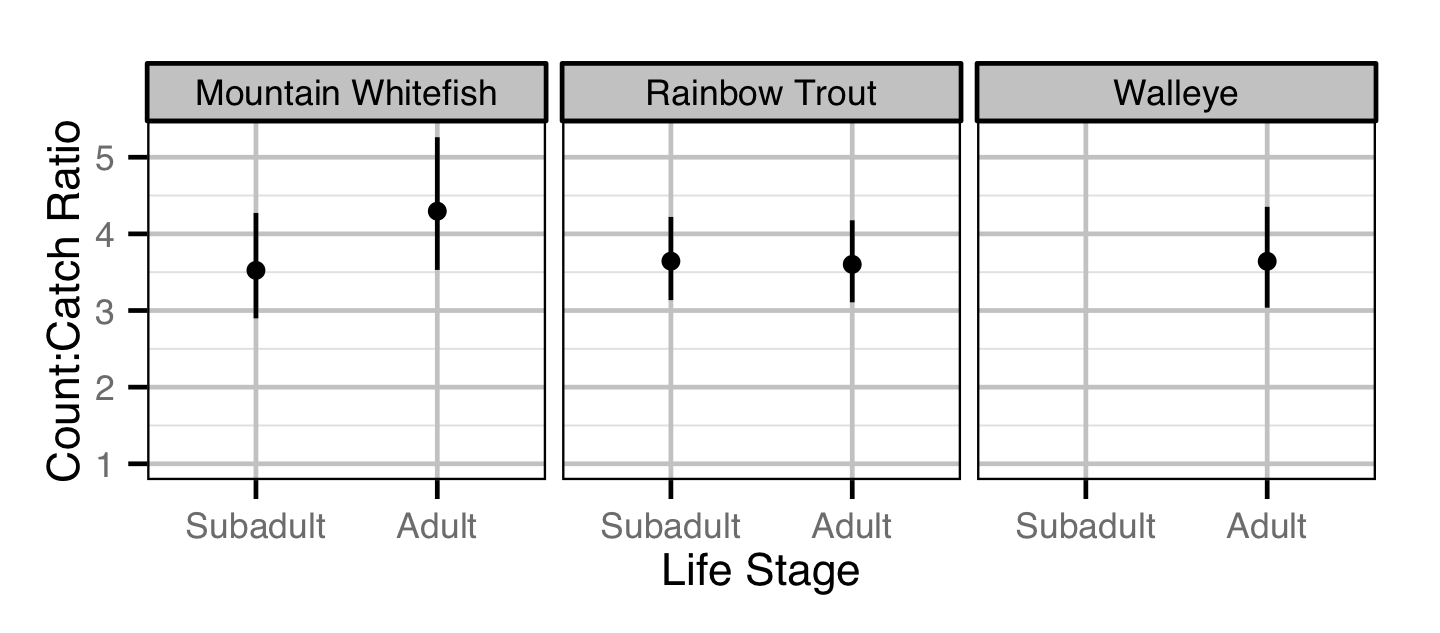
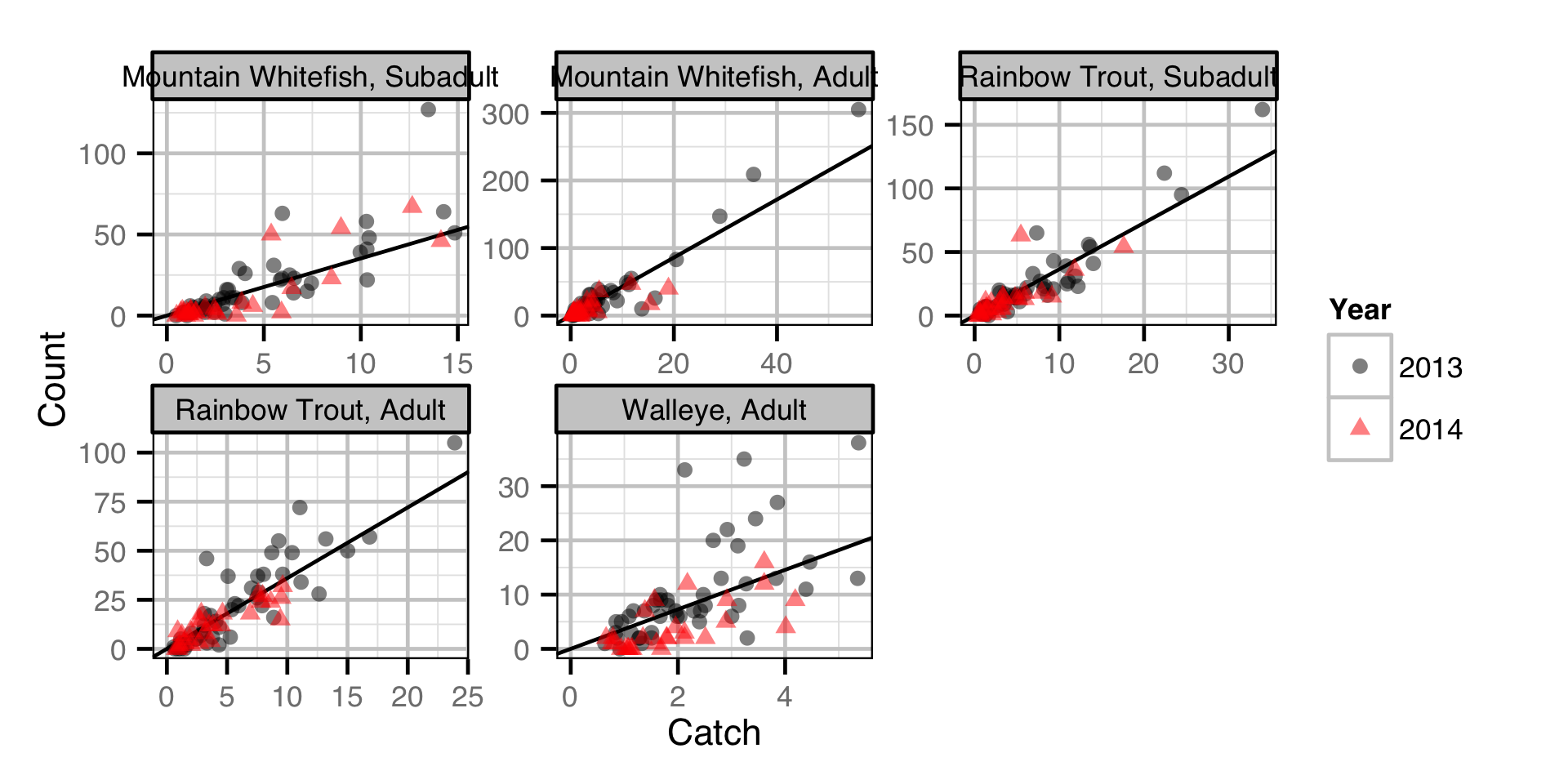
Abundance - Mountain Whitefish - Subadult
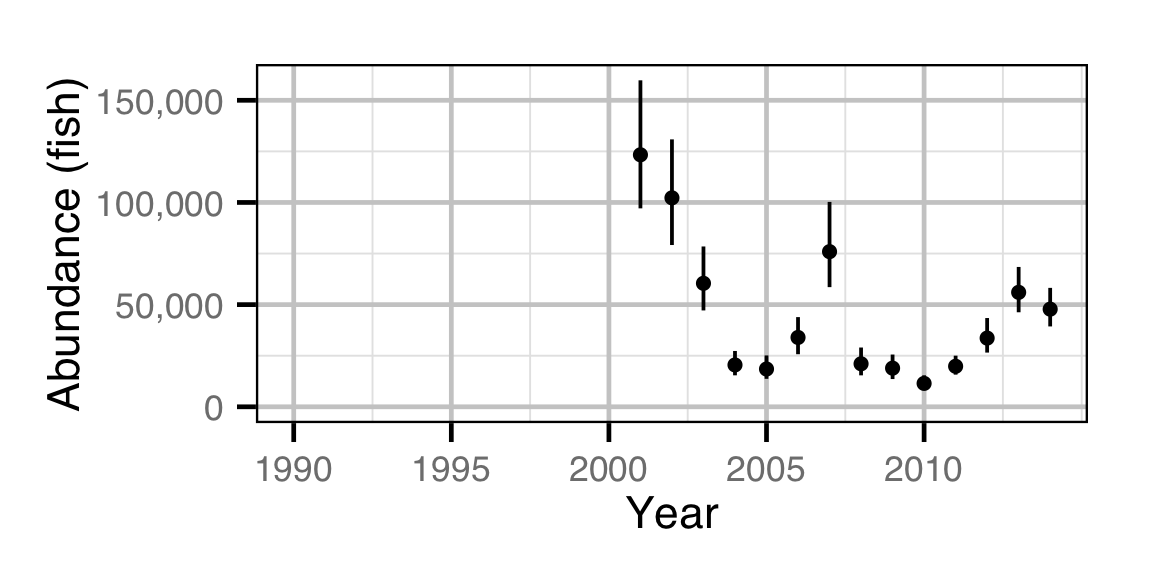
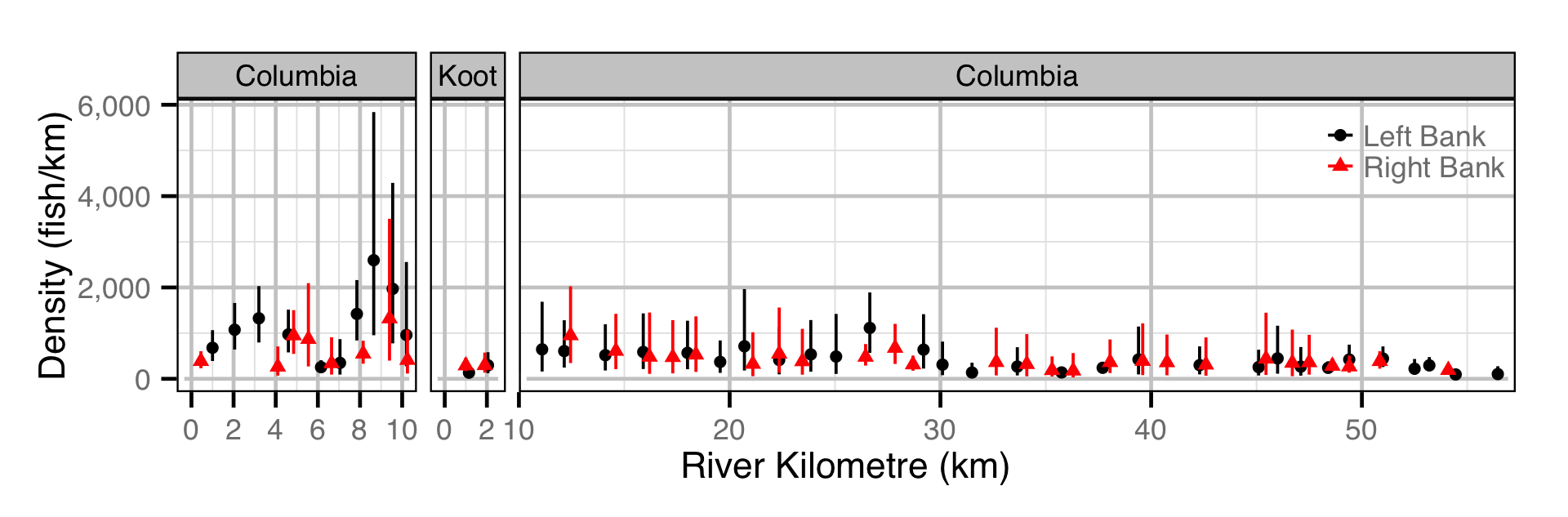
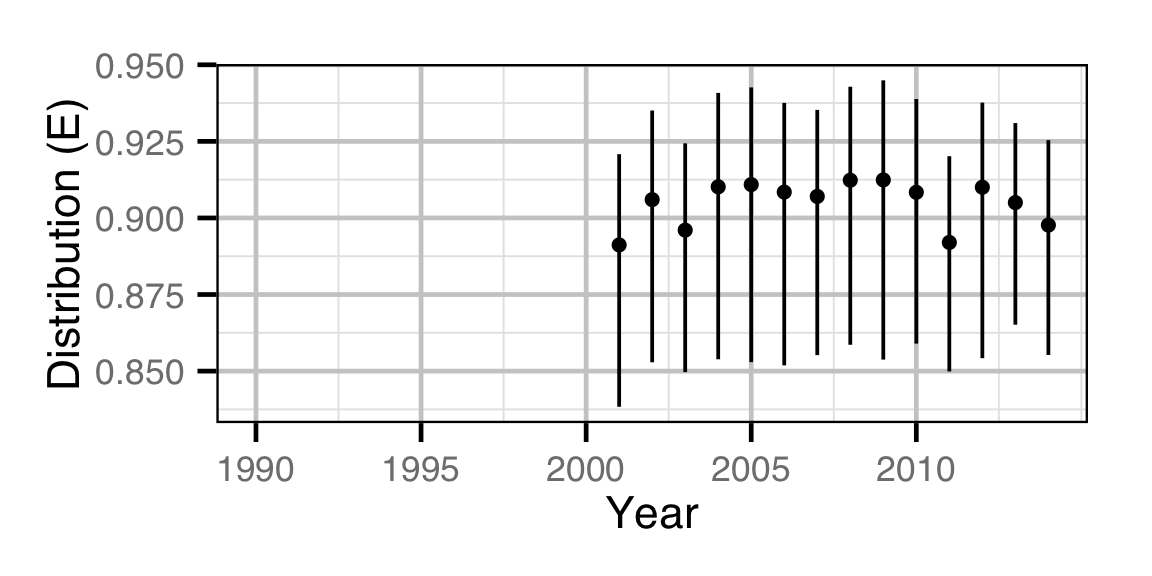
Abundance - Mountain Whitefish - Adult
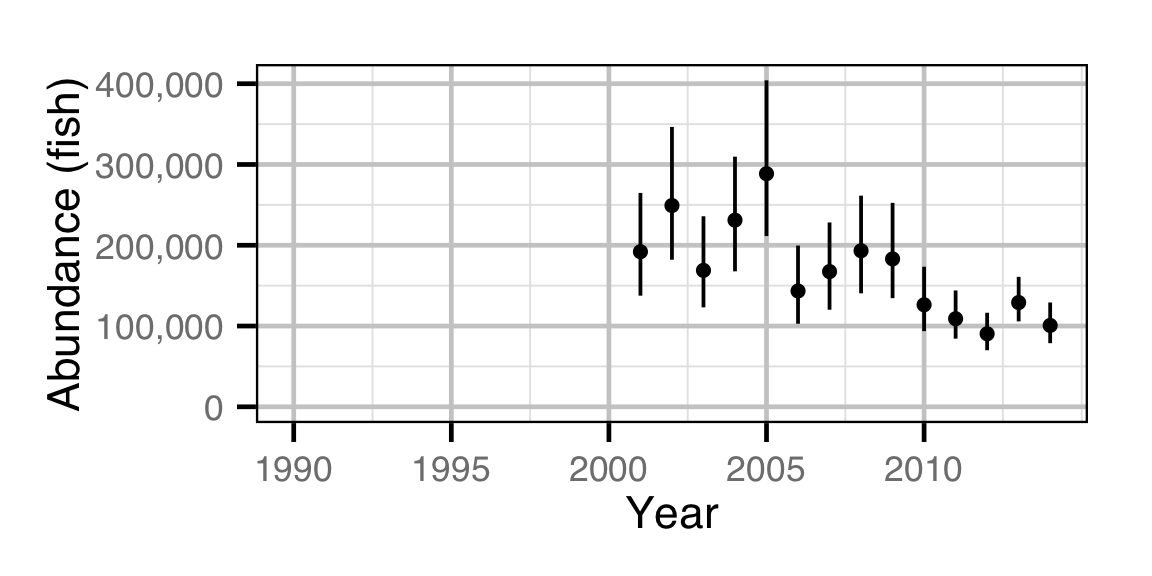
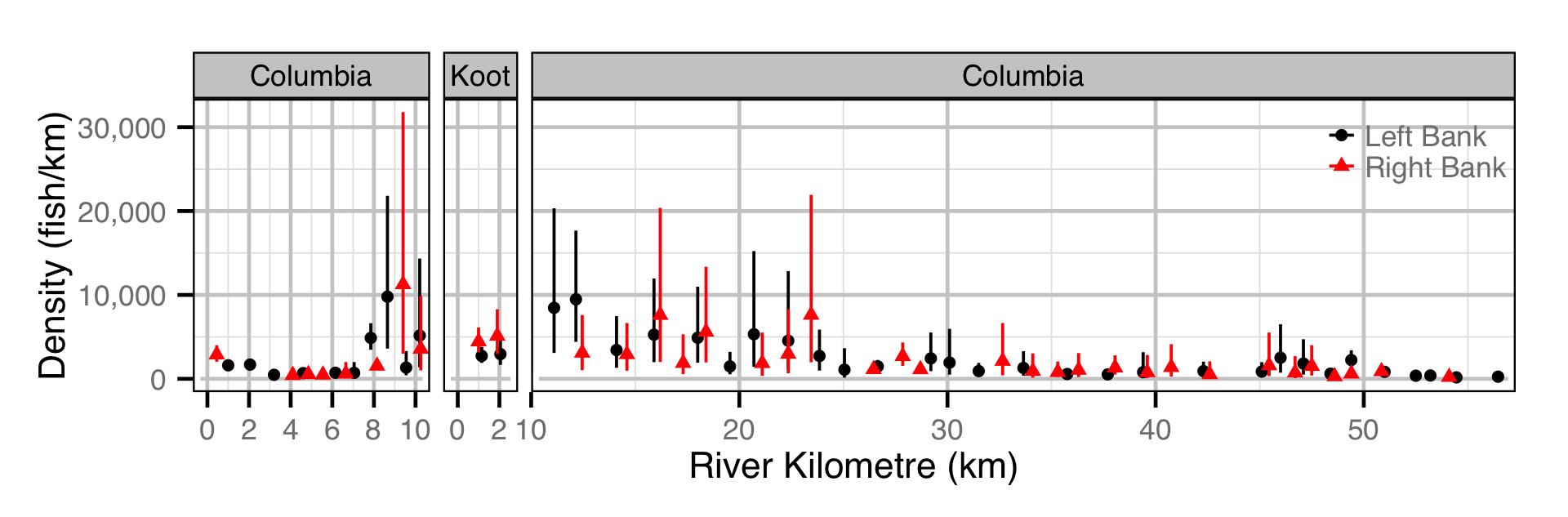
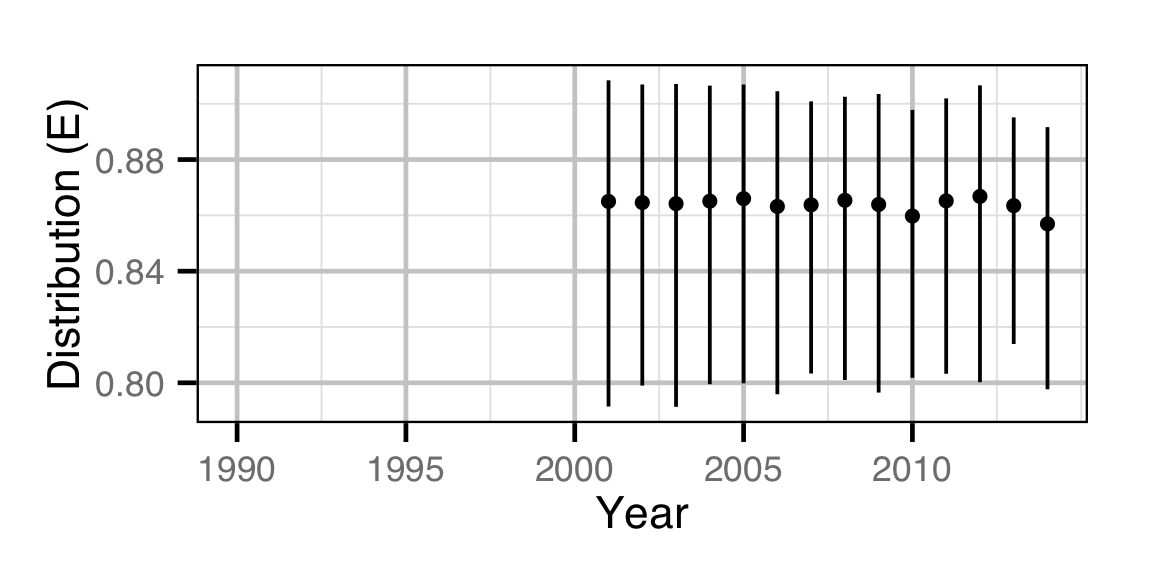
Abundance - Rainbow Trout - Subadult
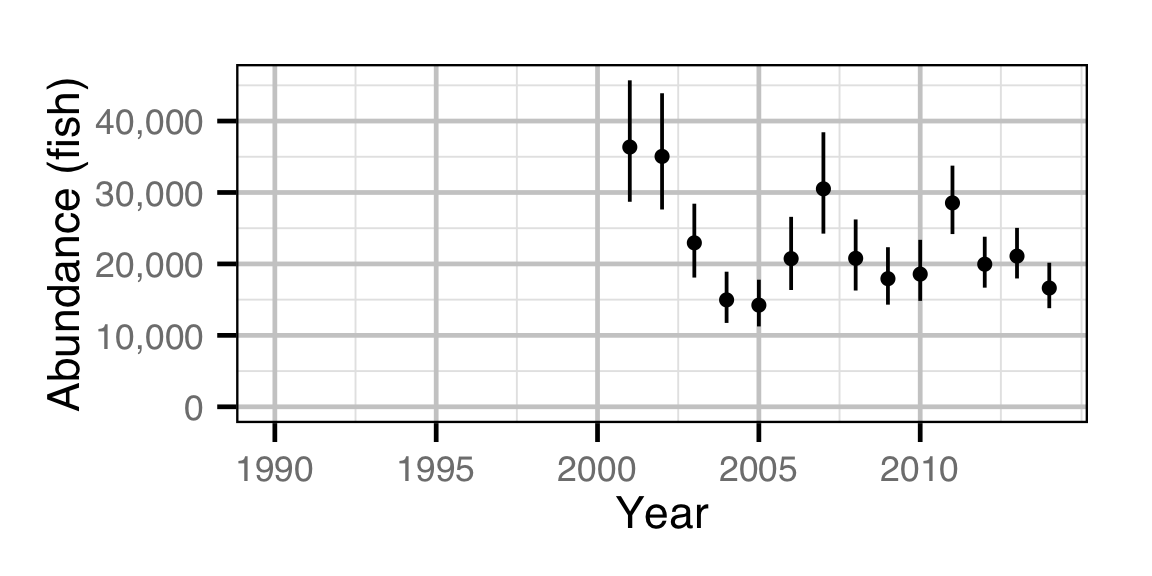
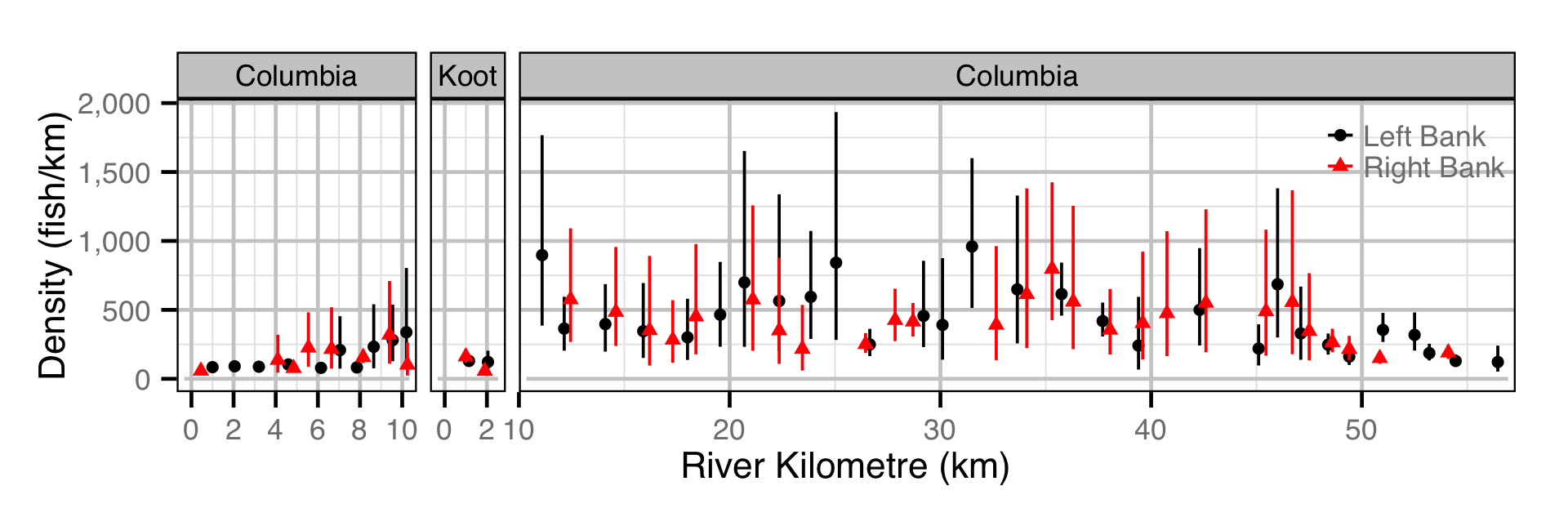
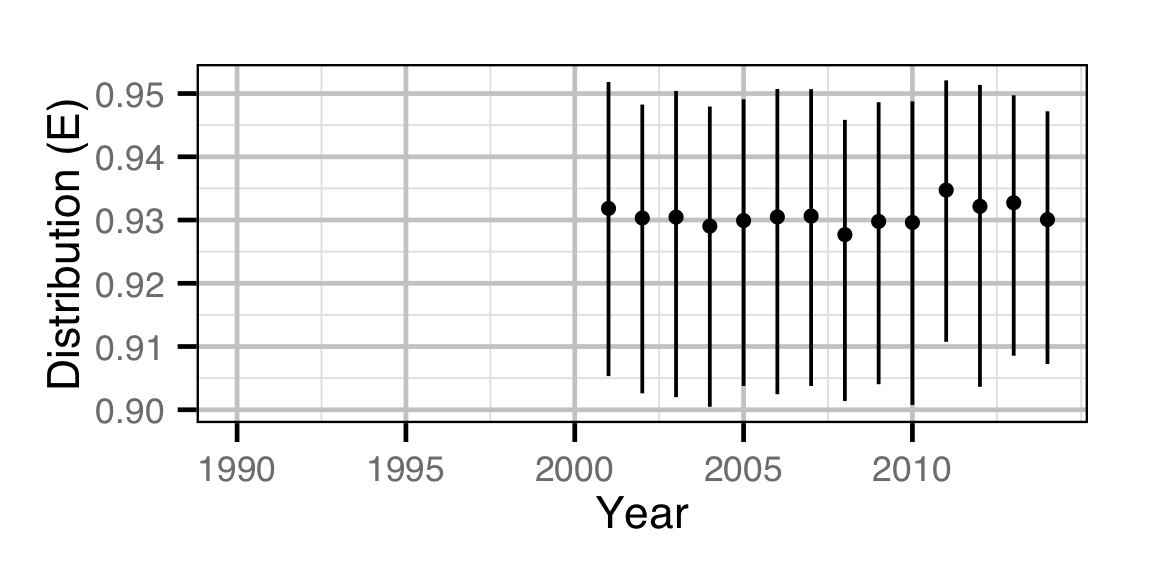
Abundance - Rainbow Trout - Adult
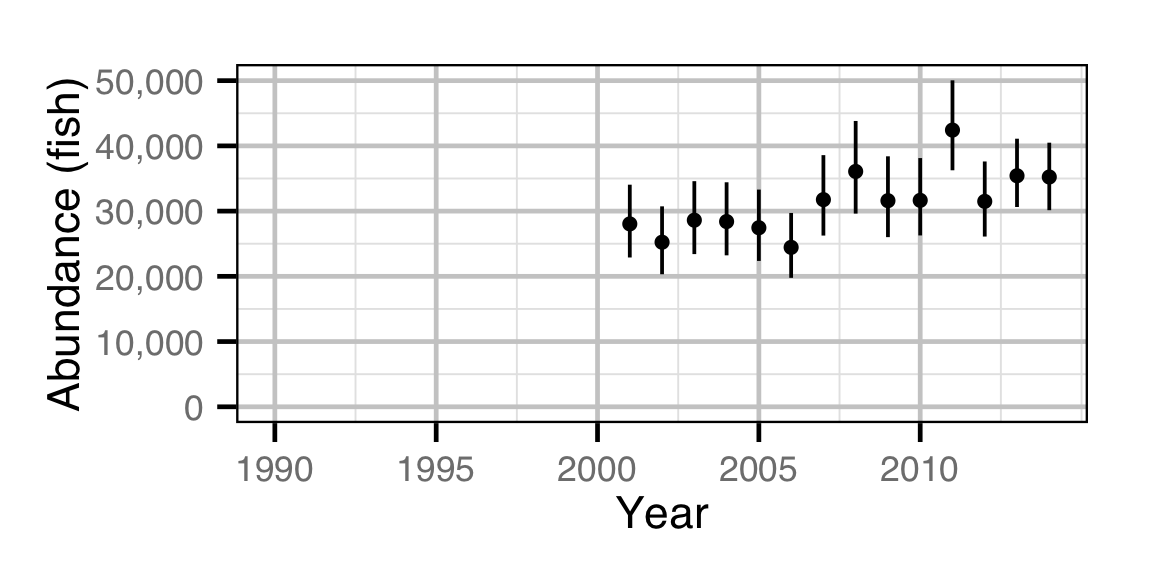
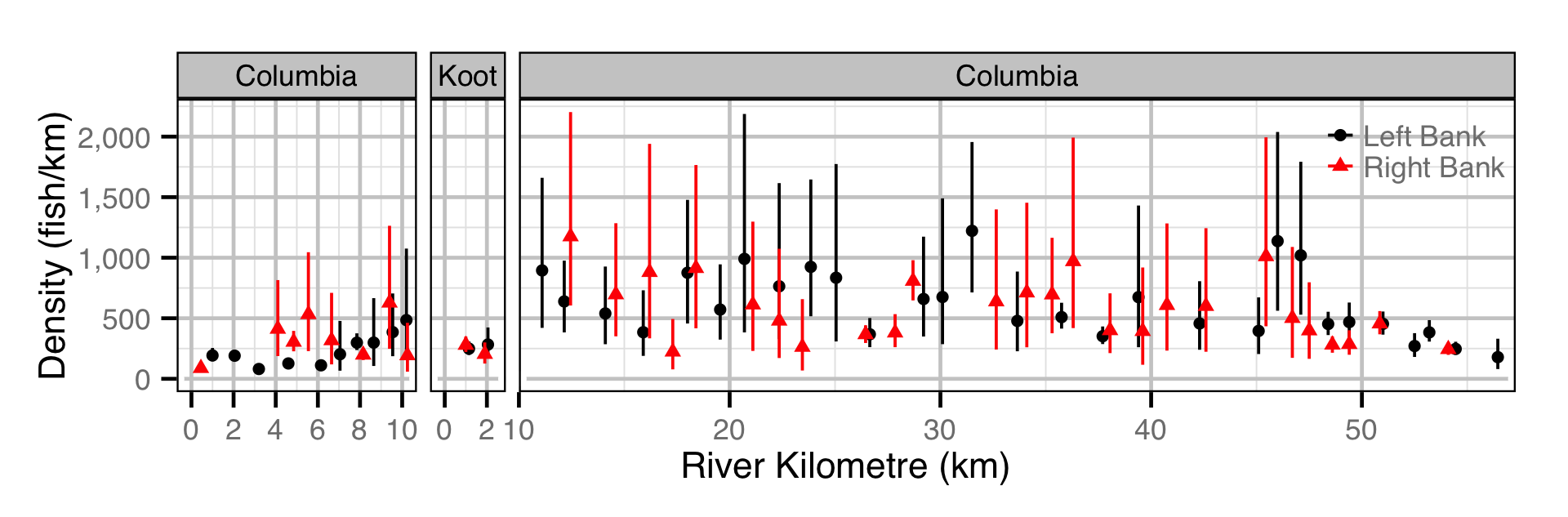
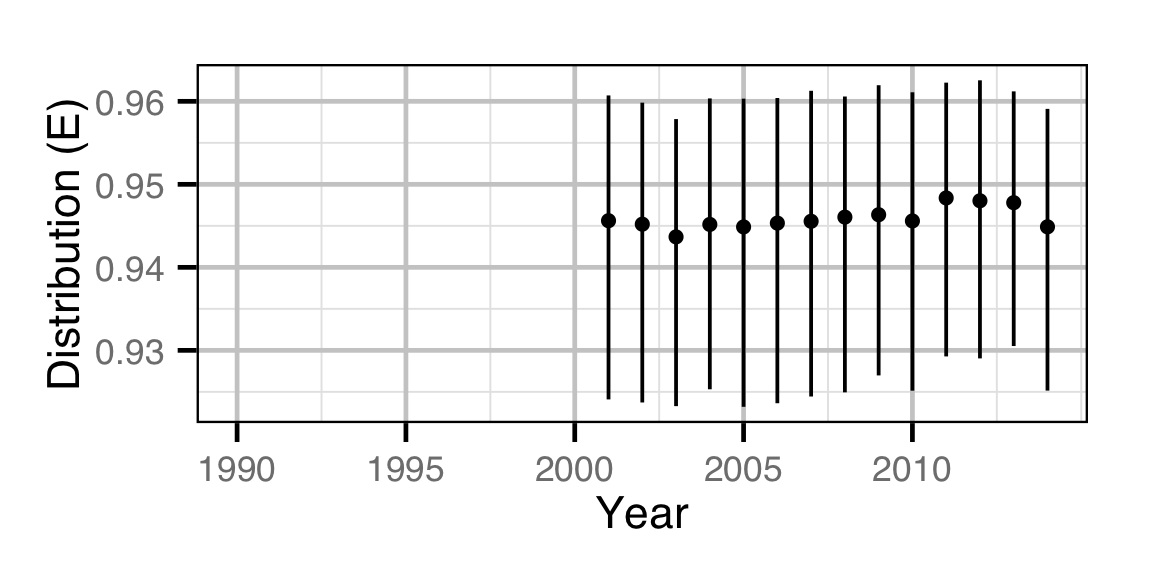
Abundance - Walleye - Adult
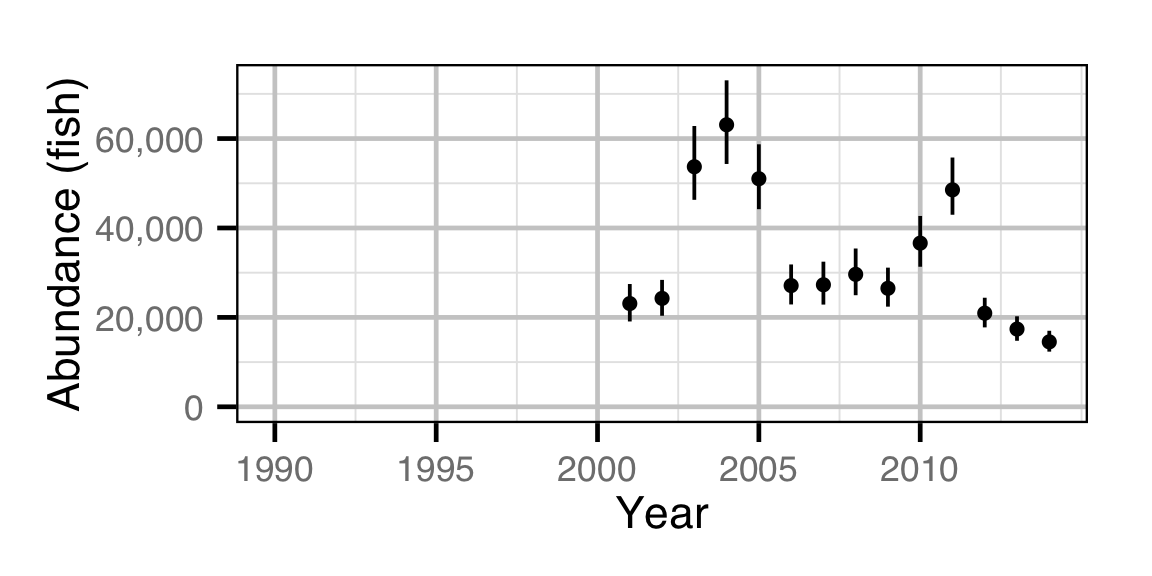
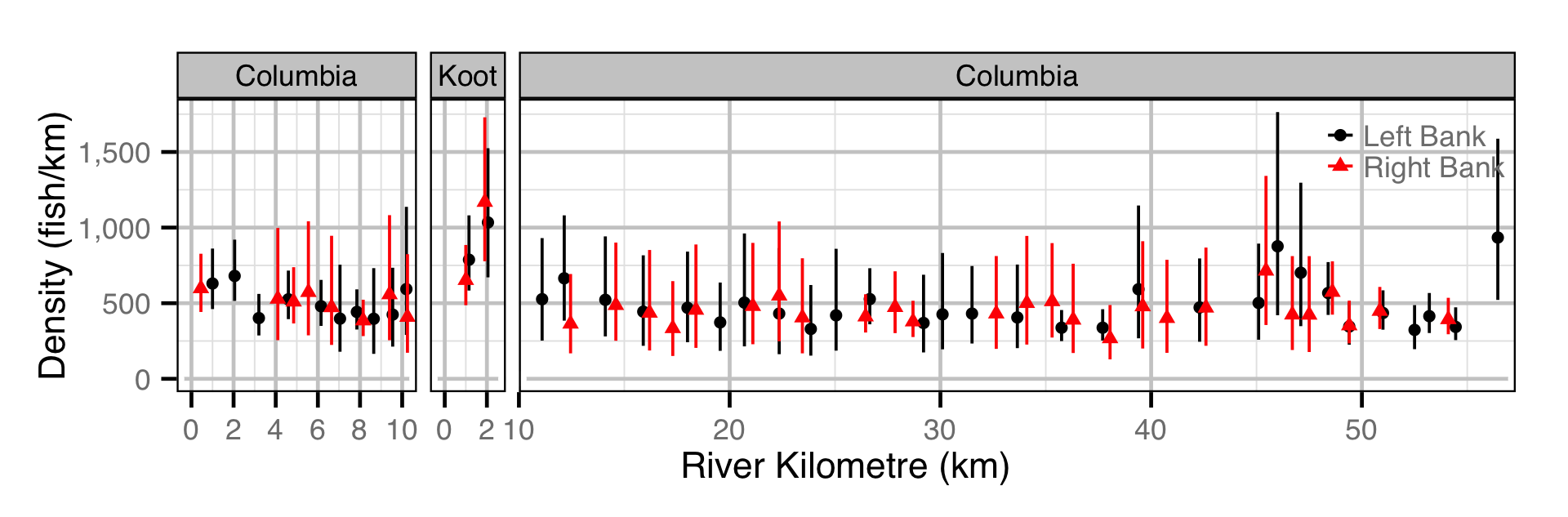
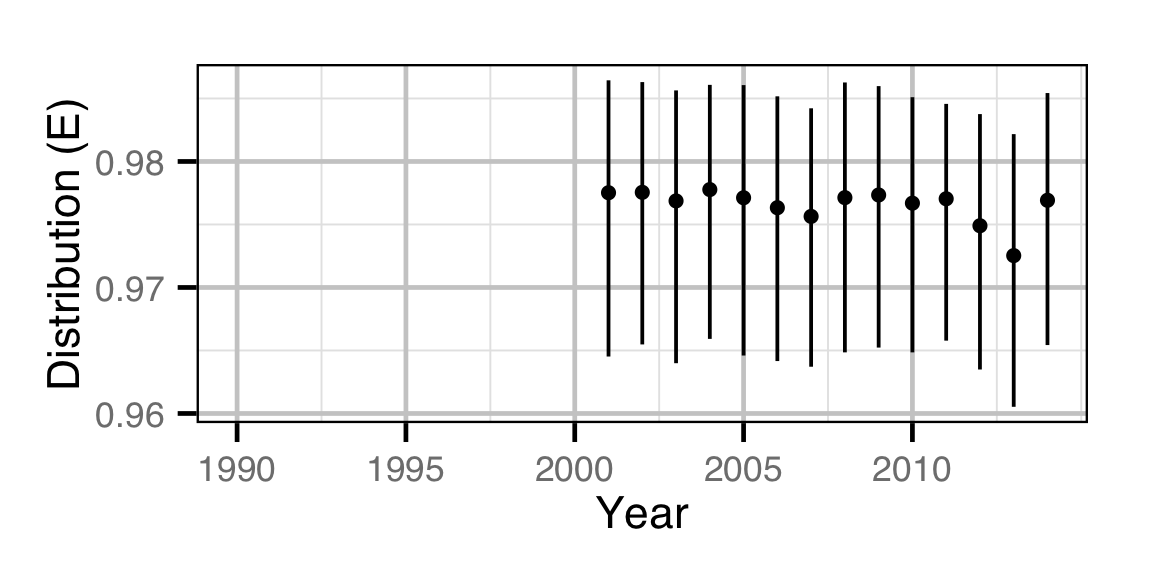
Long-Term Trends
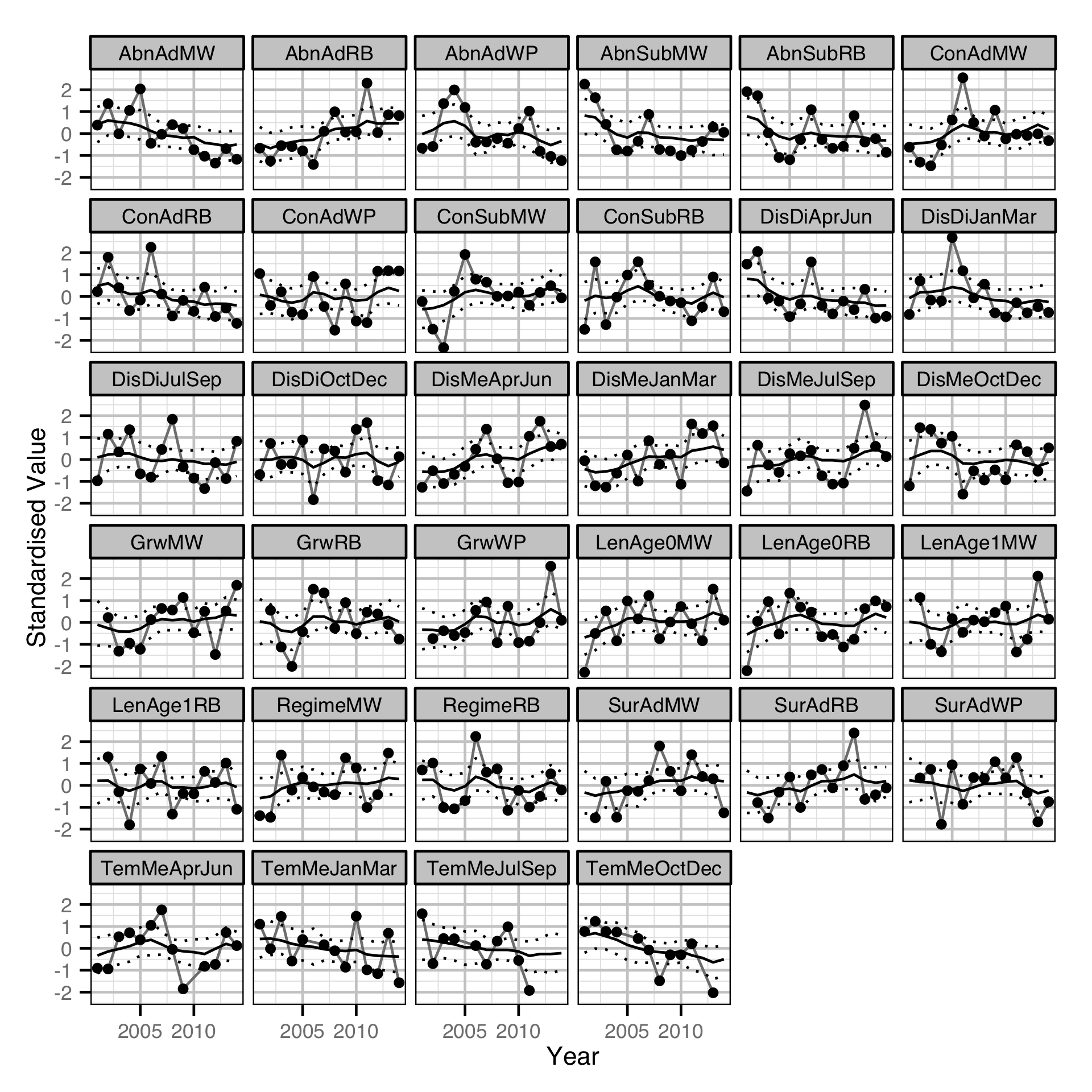
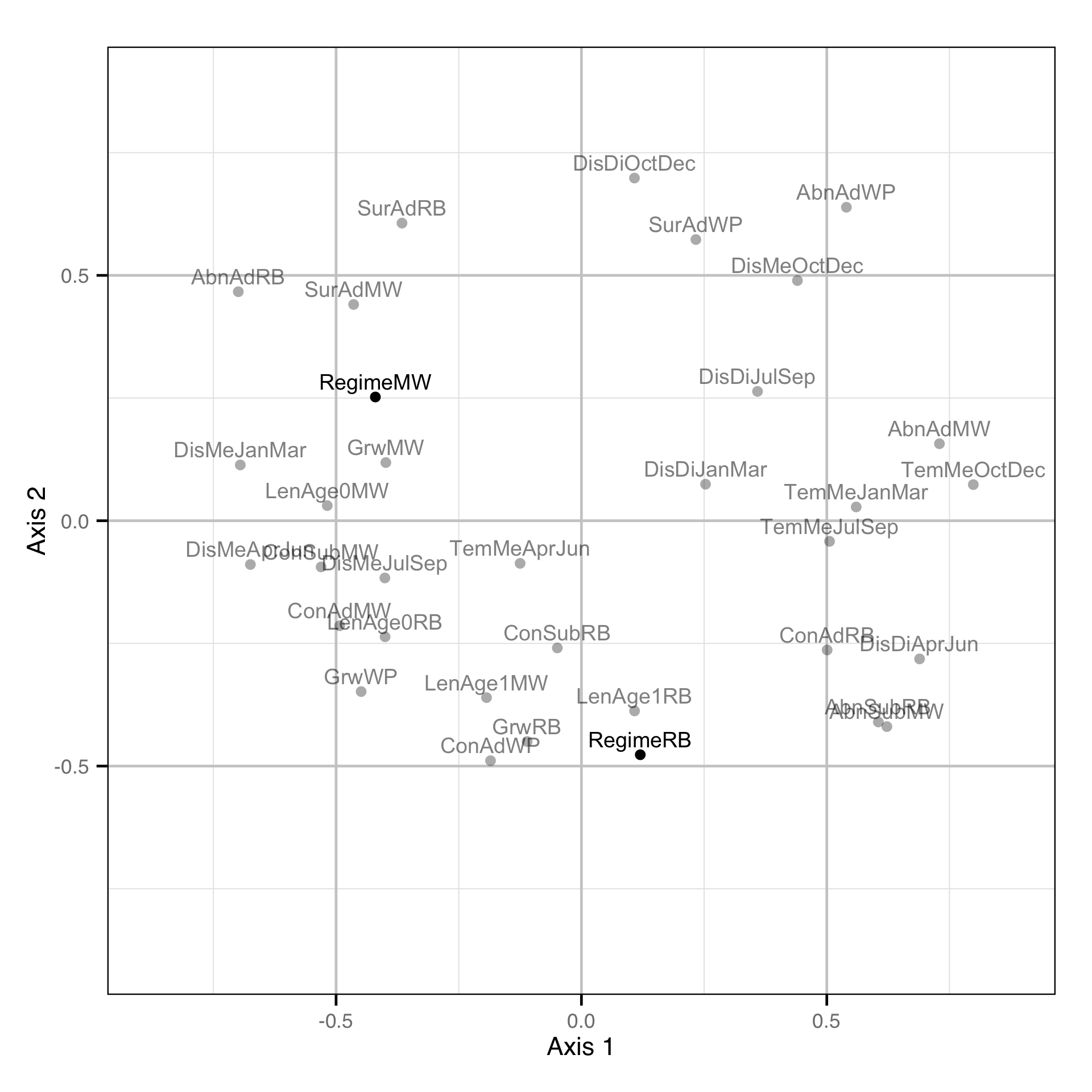
Short-Term Correlations
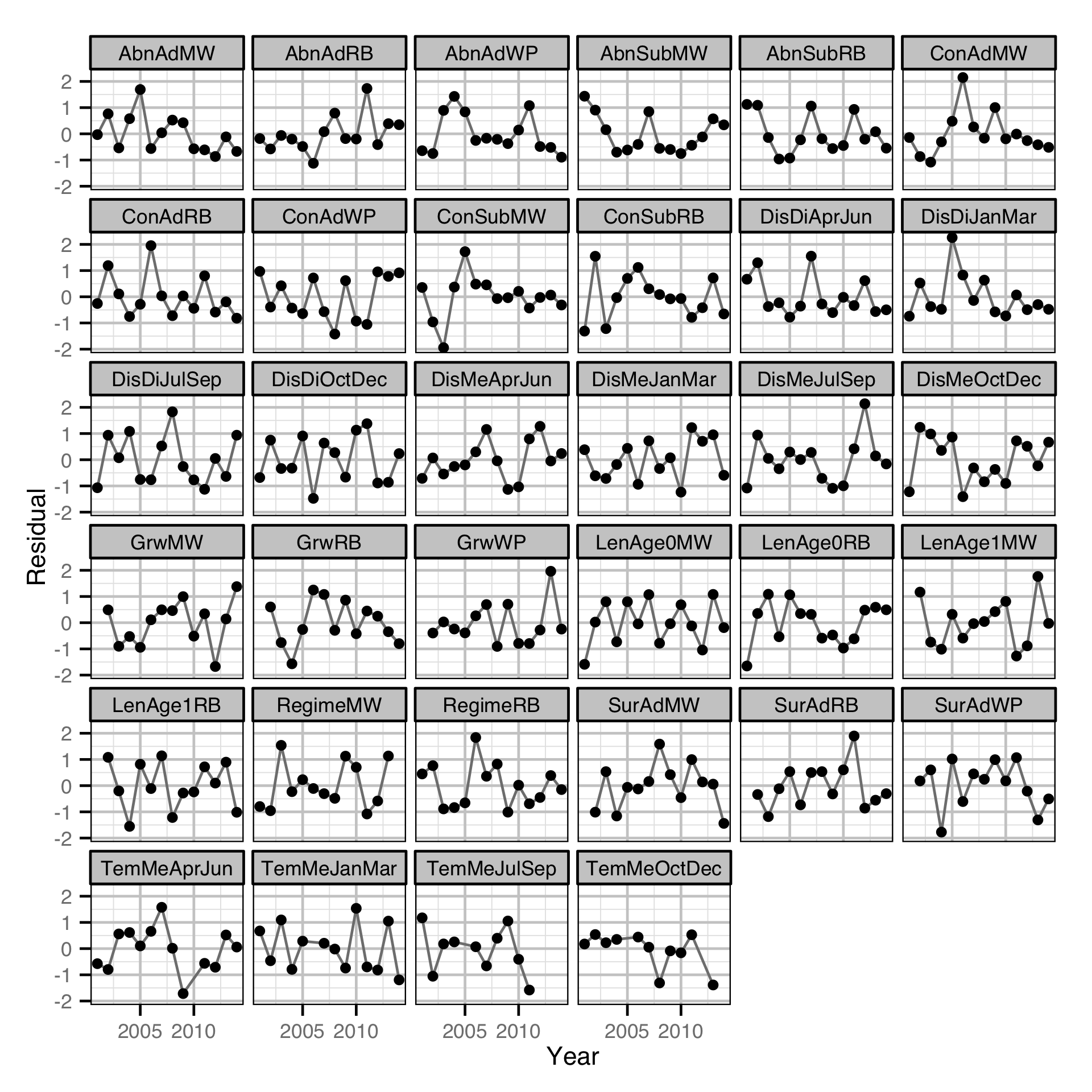
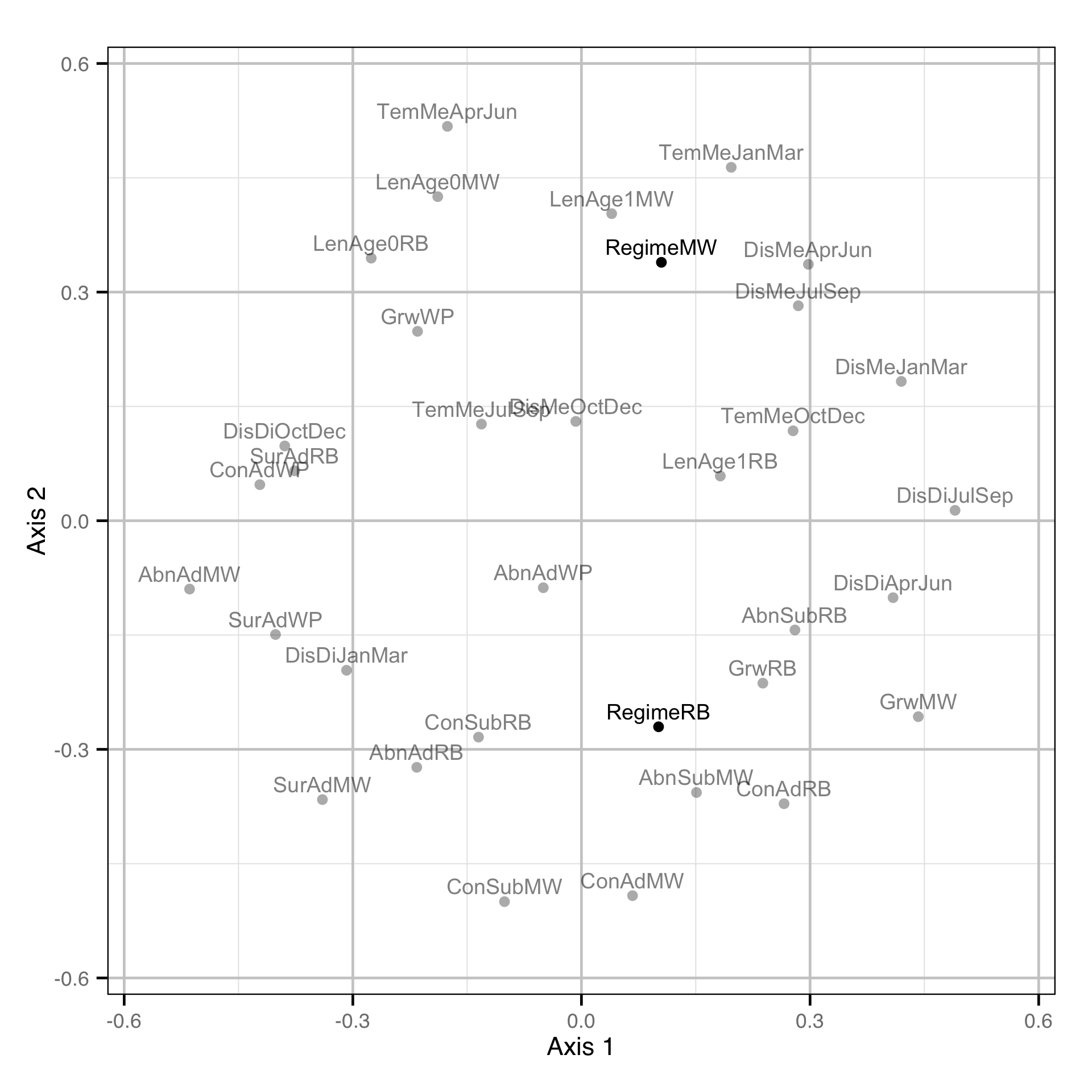
Acknowledgements
The organisations and individuals whose contributions have made this analysis report possible include:
- BC Hydro
- Golder Associates
- Demitria Burgoon
- Dustin Ford
- David Roscoe
- Sima Usvyatsov
- Dana Schmidt
- Larry Hildebrand
References
Abmann, C., J. Boysen-Hogrefe, and M. Pape. 2014. “Bayesian Analysis of Dynamic Factor Models: An Ex-Post Approach Towards the Rotation Problem.” Kiel Working Paper No. 1902. Kiel, Germany: Kiel Institute for the World Economy.
Bradford, Michael J, Josh Korman, and Paul S Higgins. 2005. “Using Confidence Intervals to Estimate the Response of Salmon Populations (Oncorhynchus Spp.) to Experimental Habitat Alterations.” Canadian Journal of Fisheries and Aquatic Sciences 62 (12): 2716–26. https://doi.org/10.1139/f05-179.
Fabens, A J. 1965. “Properties and Fitting of the von Bertalanffy Growth Curve.” Growth 29 (3): 265–89.
He, Ji X., James R. Bence, James E. Johnson, David F. Clapp, and Mark P. Ebener. 2008. “Modeling Variation in Mass-Length Relations and Condition Indices of Lake Trout and Chinook Salmon in Lake Huron: A Hierarchical Bayesian Approach.” Transactions of the American Fisheries Society 137 (3): 801–17. https://doi.org/10.1577/T07-012.1.
Irvine, R. L., J. T. A. Baxter, and J. L. Thorley. 2015. “Lower Columbia River Rainbow Trout Spawning Assessment: Year 7 (2014 Study Period).” A Mountain Water Research and Poisson Consulting Ltd. Report. Castlegar, B.C.: BC Hydro. http://www.bchydro.com/content/dam/BCHydro/customer-portal/documents/corporate/environment-sustainability/water-use-planning/southern-interior/clbmon-46-yr7-2015-02-04.pdf.
Kery, Marc. 2010. Introduction to WinBUGS for Ecologists: A Bayesian Approach to Regression, ANOVA, Mixed Models and Related Analyses. Amsterdam; Boston: Elsevier. http://public.eblib.com/EBLPublic/PublicView.do?ptiID=629953.
Kery, Marc, and Michael Schaub. 2011. Bayesian Population Analysis Using WinBUGS : A Hierarchical Perspective. Boston: Academic Press. http://www.vogelwarte.ch/bpa.html.
Macdonald, P. D. M., and T. J. Pitcher. 1979. “Age-Groups from Size-Frequency Data: A Versatile and Efficient Method of Analyzing Distribution Mixtures.” Journal of the Fisheries Research Board of Canada 36 (8): 987–1001. https://doi.org/10.1139/f79-137.
Plummer, Martyn. 2012. “JAGS Version 3.3.0 User Manual.” http://sourceforge.net/projects/mcmc-jags/files/Manuals/3.x/.
R Core Team. 2014. “R: A Language and Environment for Statistical Computing.” Vienna, Austria: R Foundation for Statistical Computing. http://www.R-project.org/.
Team, R Core. 2013. “R: A Language and Environment for Statistical Computing.” Vienna, Austria: R Foundation for Statistical Computing. http://www.R-project.org.
Thorley, J. L. 2013. “Jaggernaut: An R Package to Facilitate Bayesian Analyses Using JAGS (Just Another Gibbs Sampler).” Nelson, B.C.: Poisson Consulting Ltd. https://github.com/poissonconsulting/jaggernaut.
von Bertalanffy, L. 1938. “A Quantitative Theory of Organic Growth (Inquiries on Growth Laws Ii).” Human Biology 10: 181–213.
Zuur, A F, I D Tuck, and N Bailey. 2003. “Dynamic Factor Analysis to Estimate Common Trends in Fisheries Time Series.” Canadian Journal of Fisheries and Aquatic Sciences 60 (5): 542–52. https://doi.org/10.1139/f03-030.